Introduction
Poultry meat is popular worldwide, and chicken meat is becoming the first choice for meat consumers in lower- and higher-income countries (OECD/FAO, 2021). The global poultry meat consumption per capita is predicted to reach 15.71 kg in 2030, which represents a 5% increase from 14.97 kg in 2020 (OECD/FAO, 2022). In 2018, poultry accounted for 33% (112.86 million tons) of all meat produced (Munyaneza et al., 2021; OECD/FAO, 2021), and by 2030 it is expected to account for 41% of the total protein from meat sources; in contrast, cows, pigs and sheep are projected to contribute 20, 34, and 5%, respectively (OECD/FAO, 2021). This demonstrates that meat consumption is shifting toward poultry, likely due to its lower price compared to other meats and the increased preference for white meat (which is considered healthier) (OECD/FAO, 2021). The higher demand for poultry protein prompted the poultry industry, and particularly chicken farms, to focus on selecting and producing meat with high-quality protein and a low proportion of saturated fatty acids. In addition, chicken meat is associated with fewer cultural and religious restrictions (Liu et al., 2012; Chumngoen and Tan, 2015).
Meat quality traits play an important role in chicken farming. These traits include pH, color, drop loss, tenderness, intramuscular fat (IMF), water-holding capacity (WHC), the content and proportion of abdominal and subcutaneous fat, and flavor (Shu et al., 2010; Jung et al., 2013; Sun et al., 2013; Ono et al., 2019). IMF affects the juiciness and flavor of broiler meat (Chen et al., 2008). The taste and flavor of meat are also influenced by the nucleotide and amino acid contents (Jung et al., 2011; Hu et al., 2015). Meat is a good source of amino acids and nucleotides, and both compounds affect the taste and flavor of meat. Chicken meat, particularly breast meat, is considered healthy due to the higher protein content and lower saturated fatty acid content in comparison with meat from other livestock species, and is a source of vitamins, phosphorus, copper, iron, zinc, and other minerals (Marangoni et al., 2015; Kralik et al., 2017).
Poultry meat quality is a complex parameter; it is defined not only by intrinsic and extrinsic factors, but also by consumer preference (Ismail and Joo, 2017). Moreover, consumer preferences differ according to meat type and region. For example, lamb consumers in Australia most appreciate flavor and odor, followed by tenderness and juiciness, while for beef consumers tenderness is the most important palatability trait (Warner et al., 2010). Tenderness was reported as the most important criterion for selecting poultry products (Ismail and Joo, 2017). In another study, appearance and texture were the two most important meat quality characteristics for poultry (Fletcher, 2007). Ismail and Joo (2017) classified meat quality traits into three categories, the relative importance of which vary among consumers: appearance quality traits, such as meat color, drip loss and texture; eating quality traits, such as the tenderness, flavor and juiciness of the cooked meat; and reliance quality traits, including safety, nutrition, animal welfare, ethics, and price.
Meat quality traits are polygenic traits controlled by many genes (Gao et al., 2021). It is difficult to improve these traits using the conventional selection method (Boukha et al., 2011; Anggraeni et al., 2017), because some meat quality traits have low heritability while others have medium heritability (Ma et al., 2015). Measuring quality traits is difficult and expensive because it must be done after slaughter (Anggraeni et al., 2017). Molecular selection based on the single nucleotide polymorphisms (SNPs) of candidate genes improves meat quality traits (Van Der Steen et al., 2005; Anggraeni et al., 2017; Geng et al., 2017; Zhao et al., 2017; Gunawan et al., 2018). This review discusses genomic regions and potential candidate genes related to meat quality traits in chicken, identified through genome-wide association studies (GWAS).
Genome-wide association studies versus quantitative trait loci mapping
Identifying genes associated with polygenic traits by exploring the whole genome started in the 1990s with the quantitative trait loci (QTL) mapping approach (Soller et al., 2006; Gebreselassie et al., 2019; Habimana et al., 2021). Many QTLs for complex traits have been detected using low-density marker assays, such as microsatellites, but these are no longer applied due to low-resolution maps and large confidence intervals (Goddard and Hayes, 2009; Habimana et al., 2021). Thus, QTLs usually cover a large genomic region of potential candidate genes (Gebreselassie et al., 2019; Habimana et al., 2021). GWAS are preferred over QTL as they represent a more precise genomic approach to detect causal variants in narrow genomic regions (Hirschhorn et al., 2005; Gebreselassie et al., 2019; Habimana et al., 2021).
GWAS can show the relationship between the variant and trait of interest, and do not make assumptions regarding the effects of the QTLs or candidate genes on traits (Sharmaa et al., 2015; Berghof et al., 2018; Ji et al., 2019; Habimana et al., 2021). GWAS are effective and powerful genetic approaches to detect genomic regions and potential candidate genes related to traits of interest (Raza et al., 2020); they focus on the most significant SNPs, and ignore those of less significance even when they are relevant in terms of biological function (Do et al., 2014). This genomic approach was applied herein to different livestock species, including chickens, to detect potential candidate genes and SNPs associated with meat quality traits.
Studies have used different software and statistical models to identify SNPs significantly associated with traits of interest. Habimana et al. (2021) used a mixed linear model (MLM) of Tassel 5.2.60 to identify SNPs associated with the body weight of indigenous chickens in Rwanda via GWAS. Additionally, Liu et al. (2013) used a simple general linear model (GLM) and compressed MLM to identify SNPs significantly associated with body composition and meat quality traits in Beijing-You chickens. This compressed MLM was also used by Sun et al. (2013) to identify the associations between SNPs and meat quality traits in Beijing-You chickens crossed with Cobb broilers.
In contrast, QTL analyses employing different statistical models have been performed to identify SNPs significantly associated with traits of interest in chickens. For example, Pampouille et al. (2018) used a univariate linear model to identify SNPs significantly associated with white striping related to breast muscle yield and meat quality traits in broiler chickens. Jia et al. (2012) used the SAS program (SAS Institute, Cary, NC, USA) to identify SNPs significantly associated with body weight, fatness and body composition in chickens, while Jin et al. (2020) used an MLM to identify SNPs significantly associated with fatty acid composition in the breast and thigh of Korean native chickens.
Application of GWAS to chickens
GWAS have already been carried out on growth (Gu et al., 2011; Xie et al., 2012; Sun et al., 2013), egg production and quality (Liu et al., 2011; Sun et al., 2013), disease resistance (Fife et al., 2011; Sun et al., 2013), and meat quality traits in chickens (Sun et al., 2013). The meat quality traits considered in the present review are dry matter content, meat color, skin color, abdominal fat, pH, drop loss, tenderness, IMF, fatty acid composition and nucleotide and amino acid contents. The current review summarizes new knowledge about potential candidate genes for meat quality traits in chicken.
Candidate genes for intramuscular fat content
Meat is a source of protein, minerals, essential fatty acids, including linoleic and alpha-linolenic acids, and lipid-soluble vitamins (A, D, E, and K) (Marangoni et al., 2015; Kralik et al., 2017). Higher fat intake, particularly saturated fatty acids, has been associated with cardiovascular disease and cancers ( and , 2017). Chicken meat has low saturated fatty acid content and relatively high polyunsaturated fatty acid content (Maharani et al., 2013).
Fat content affects meat quality and consumer acceptance due to its effect on the taste, smell and texture of food, including chicken meat (Marangoni et al., 2015). Fat content in chicken varies depending on the cut. IMF affects the sensory characteristics of poultry meat, such as juiciness, flavor and tenderness (Zhao et al., 2007; Chen et al., 2008; Hocquette et al., 2010; Ismail and Joo, 2017). GWAS of chickens identified various candidate genes associated with fat and IMF in chickens, including CCK, TOLLIP, MGST1, NTPCR, AGA, TYRO3 and KIF2A (Table 1).
The cholecystokinin (CCK) gene is important for the storage of triglycerides in adipose tissue ( et al., 2021). It is also involved in the regulation of gallbladder contraction and pancreatic secretion ( et al., 2021). The CCK gene plays a major role in the digestion of fat (Chandra and Liddle, 2007; Liu et al., 2013). The Toll-interacting protein (TOLLIP) gene plays a role in innate immunity, as well as the development of atherosclerosis and lung diseases (Li et al., 2021). The microsomal glutathione S-transferase 1 (MGST1) gene of chickens encodes a protein that is involved in reducing lipid hydroperoxides (Sun et al., 2013).
The nucleoside-triphosphatase, cancer-related (NTPCR) gene is involved in the formation of tumors in humans (Placzek et al., 2007; Sun et al., 2013). In chickens, aspartylglucosaminidase (AGA), AXL receptor tyrosine kinase (TYRO3) and kinesin family member 2A (KIF2A) genes have been mapped to chromosomes 4, 5, and Z, respectively. TYRO3 has a role in cell proliferation and differentiation (Rizkalla et al., 2012; Sun et al., 2013). The AGA gene encodes the enzyme AGA, which is involved in the breakdown of glycoproteins in lysosomes (Uusitalo et al., 1997). The protein encoded by the KIF2A gene is necessary for normal brain development (Broix et al., 2018).
Candidate genes for ultimate pH
The pH of muscle and WHC affect the sensory characteristics of meat products (Chung et al., 2015). The sensory characteristics of meat include color, flavor, juiciness and tenderness ( and , 2015). Two types of pH are measured in meat: the initial pH, which is the pH measured 45 min post-mortem, and the ultimate pH (pHu), which is measured 24 hours post-mortem (Davoodi and Ehsani, 2020). In chicken meat, pH values 15 min after slaughter are between 6.2 - 6.5 (Berri et al., 2005; Ismail and Joo, 2017), and a normal pHu is about 5.8 (Ismail and Joo, 2017). When the pH value is < 5.8, the myofibrillar protein decreases WHC, and discoloration of the meat occurs; the lower WHC also affects drip loss (Ismail and Joo, 2017). GWAS detected some genes potentially related to pH, including the GALNT, PCDH19, DIAPH and SPP2 genes (Table 1).
The polypeptide N-acetylgalactosaminyltransferase 1 (GALNT1) gene is involved in the glycosylation process, which is a common post-translational protein-modifying process important for the stability and secretion of signaling molecules (Sellers et al., 2008; Liu et al., 2013). The SNP (SNP rs17647532) of the GALNT1gene is associated with ovarian cancer in humans (Sellers et al., 2008). The protocadherin19 (PCDH19) gene encodes the protocadherin19 protein, which helps cells in the brain communicate. Mutations in the PCDH19 gene are associated with epilepsy (Tan et al., 2018; Yang et al., 2020). The diaphanous-related formin 1 (DIAPH1/DIAPH2) gene, also known as diaphanous-related formin 1 (DIAPH1), encodes a protein that plays a role in the occurrence and development of tumors in humans ( et al., 2021). The secreted phosphoprotein 2 (SPP2) gene is involved in bone metabolism (Liu et al., 2015).
Candidate genes for inosine 5′-monophosphate
Previous studies reported a significant effect of nucleotide and amino acid contents on meat quality traits in chickens. Meat flavor is the most important characteristic of meat quality, and is affected by nucleotides such as inosine 5′-monophosphate (IMP) (Jung et al., 2011; Qin et al., 2011; Jung et al. 2013), and amino acids including asparagine, threonine, serine, glutamate and glycine (Jung et al., 2011; Hu et al., 2015). IMP content is used as a criterion of meat flavor and freshness in many countries (Chen et al., 2002). The decomposition of IMP produces inosine and hypoxanthine (Uemoto et al., 2017). The nucleotide and amino acid contents of chicken meat depend on breed, age, sex and cooking method (Jung et al., 2013; Jayasena et al., 2015; Jin et al., 2017). A few genes have been shown to be associated with IMP in chicken meat, such as the ADSL, AMPD1, ATIC, GPAT, AIRC, and PURH genes (Shu et al., 2010; Hu et al., 2015; Ma et al., 2015). However, no GWAS of nucleotide and amino acid contents in chickens have been published. Genes potentially linked with IMP are presented in Table 1.
Candidate genes for meat and skin color
Meat color is the most important appearance quality trait, as it indicates the freshness of the product and influences consumer acceptance of poultry meat (Qiao et al., 2001; Haraf et al., 2009; Joo et al., 2013; Ismail and Joo, 2017). Undesirable meat color leads to rejection without even examining other meat qualities, such as tenderness, flavor, texture and taste (Ismail and Joo, 2017). Poultry meat color is affected by genetic and environmental factors, such as sex, age, strain, processing technology and storage (Fletcher et al., 2000). The difference in concentration of myoglobin among the different parts of poultry is responsible for the different colors of poultry meat, ranging from the dark-colored leg and thigh (with relatively high myoglobin content) to the whitish breast meat (Wideman et al., 2019). GWAS detected potential candidate genes related to meat and skin color in chicken, such as the COL1A2, KPNA2, PSMD12, FTSJ3, PTPN2, SPINK5, CLINT1, PGD, MIR1587, and BUB3 genes (Table 2).
The collagen type I alpha 2 chain (COL1A2) gene has SNP (rs414408) that is significantly associated with dental fluorosis, while other mutations are significantly associated with diseases of the bone, cartilage and blood vessels (Ibiyemi et al., 2020). In the chicken genome, the karyopherin subunit alpha 2 (KPNA2) gene encodes for the karyopherin alpha 2 proteins, which play a major role in many cancers such as lung, ovarian, and prostate cancers, among many others ( et al., 2013). The proteasome 26S subunit non-ATPase 12 (PSMD12) gene has been associated with a neurodevelopmental disorder ( et al., 2018). In chicken, the FtsJ3 homologous gene significantly promotes breast cancer in humans (Manning et al., 2020). The candidate genes potentially associated with the meat and skin color of chicken are presented in Table 2.
Candidate fatty acid composition genes
Meat consumers desire healthier meat. Fatty acid composition affects meat quality due to its contribution to sensory qualities; it also affects human health (Van Son et al., 2017). Fatty acids are components of fats subdivided into saturated and unsaturated fatty acids (Hidayati et al., 2015).
Higher consumption of fatty acids, particularly saturated fatty acids, has been linked with cardiovascular disease (Maharani et al., 2012). However, unsaturated fatty acids reduce the risk of cardiovascular disease (Milićević et al., 2014). The predominant fatty acids in chicken are palmitic acid, stearic acid (saturated fatty acids), and oleic acid (unsaturated fatty acid) (Milićević et al., 2014; Tougan et al., 2018). Factors such as genotype, age, gender, temperature, feeding and muscle type affect the fatty acid profile of chicken meat (Trembecká et al., 2016; Tougan et al., 2018).
Based on the accession date of February 2022, 464 QTLs associated with different meat quality traits were identified in chickens (Animal QTL database). Among the QTLs detected, only a few are related to fatty acid traits. The reasons for the shortage of information on QTLs related to fatty acid composition in chickens were discussed by Jin et al. (2018) and include the large effect of environmental factors on the composition of chicken meat and the lower content of fat in chicken meat. Jin et al. (2020) identified different QTLs associated with fatty acid composition in chicken meat, such as a QTL identified on chromosome 23 (ID: 193112) associated with docosahexaenoic acid (C22:6). A QTL identified on chromosome 18 (ID: 19311) has been associated with linolenic acid (C18:3), one identified on chromosome 6 (ID: 193106) has been linked to oleic acid (C18:1) content, and another on chromosome 18 (ID: 193110) has been linked to palmitic acid (C16:0) and linoleic acid (C18:2). A QTL identified on chromosomes 4, 6, and 18, and found on chromosome 6, has been linked with stearic acid (C18:0). Various potential candidate genes for fatty acid composition in chicken have been reported, such as the DEGS1, ELOVL6, FABP3, FABP4, FASN, and SCD genes (Jin et al., 2020). However, no GWAS have been carried out for fatty acid composition in chickens. The candidate genes associated with fatty acid composition in chicken are summarized in Table 3. In chickens, potential candidate genes for different meat quality traits are mapped on different chromosomes. The locations of the genes potentially related to meat quality traits in chicken are summarized in Fig. 1.
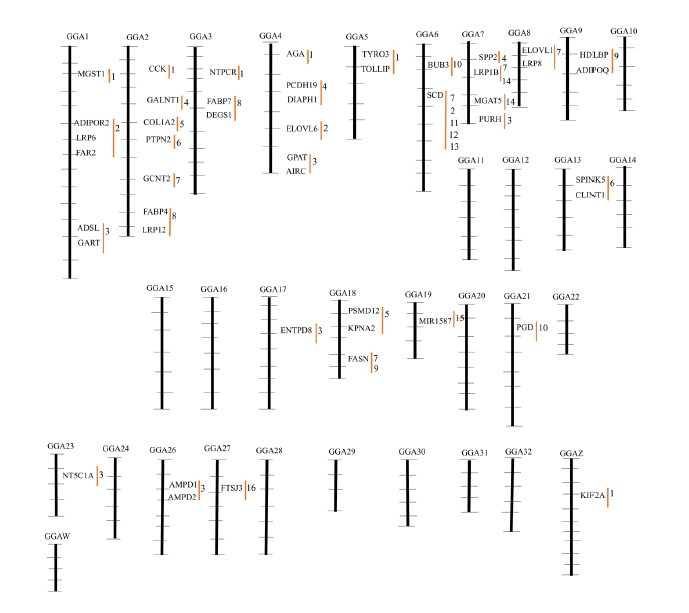
Fig. 1. Locations of the potential candidate genes related to meat quality traits in chicken. 1Intramuscular fat (IMF), 2linoleic acid (C18:2), 3inosine 5’-monophosphate (IMP), 4ultimate pH (pHu), 5meat lightness (Meat L*), 6skin lightness (Skin L*), 7palmitic acid (C16:0), 8eicosenoic acid (C20:1), 9linolenic acid (C18:3), 10skin yellowness (Skin b*), 11stearic acid (C18:0), 12oleic acid (C18:1), 13palmitoleic acid(C16:1), 14arachidonic acid (C20:4), 15skin redness (skin a*), 16meat yellowness value (meat b*).
Conclusion
GWAS are reliable genomic approaches to identify variation in meat quality traits of chickens. Various candidate genes potentially associated with meat quality traits in chicken have been detected by GWAS. However, there is a lack of literature regarding GWAS for fatty acid composition traits, nucleotides and amino acid contents, which also affect chicken meat quality. Moreover, many chicken breeds, particularly native breeds need to be considered due to their superior qualities. Therefore, it is essential to continue researching meat quality traits via GWAS, to discover more genes related to important meat quality traits to meet consumer preferences.
Acknowledgements
This work was supported by the grant number PJ0162052022 of the Rural Development Administration of Korea.
Authors Information
Jean Pierre Munyaneza, https://orcid.org/0000-0003-2521-3339
Thisarani Kalhari Ediriweera, https://orcid.org/0000-0001-9649-6925
Minjun Kim, https://orcid.org/0000-0002-8173-8431
Eunjin Cho, https://orcid.org/0000-0003-4800-1603
Aera Jang, https://orcid.org/0000-0003-1789-8956
Hyo Jun Choo, https://orcid.org/0000-0002-7747-5077
Jun Heon Lee, https://orcid.org/0000-0003-3996-9209