Introduction
Medicinal plants have been used for thousands of years in developing countries. According to the World Health Organization (WHO), traditional healthcare systems serve 70 - 80% of the population in Africa, India, and other developing countries (Kumar and Jnanesha, 2016). The Indian state of Uttarakhand, also known as the herbal state, is a reservoir of MAPs. Traditional uses for MAPs included the treatment of leukorrhea, diabetes, kidney stones, kidney disease, cuts, wounds, fever, jaundice, stomach issues, rheumatism, and kidney stones. The industry is once again on the rise as a result of the recent significant increase in the use of natural essential oils. India is one of the few countries that can produce a majority of the essential oils required by the pharmaceutical, flavoring, and cosmetic industries (Chandra et al., 2022). Essential oils are complex mixtures of secondary metabolites that include phenylpropenes and terpenes with low boiling points (Greathead, 2003). Essential oils have distinct flavor and fragrance properties, as well as biological activities, and are widely used in aromatherapy and healthcare, as well as in cosmetics, flavorings and fragrances, spices, pesticides and repellents, and herbal beverages. Aromatic plants' antioxidant and antimicrobial activities have been extensively researched and were found to have health applications in the prevention and reduction in the risk of diseases such as inflammation, atherosclerosis, cardiovascular disease, and cancer (Gutteridge and Halliwell, 2010; Ndhlala et al., 2010). Various plant families, such as Asteraceae, Lamiaceae, Myrtaceae, Rutaceae and Verbenaceae are well known among essential oil containing plant families. These families possess various therapeutic properties and both traditional and modern uses (Samarth et al., 2017; Michel et al., 2020; Kholiya et al., 2022). Various distillation processes/methods such as gas chromatography–mass spectrometry (GCMS) (Meng et al., 2014), high performance liquid chromatography (HPLC) (Porel et al., 2014), and hydro distillation (Irshad et al., 2020) are commonly used methods for the separation of essential oils from aromatic plant materials. These techniques enable the highest detection limits, but real-time examination of essential components in plants is very difficult due to their time-consuming, destructive, and complex sample preparation characteristics. Therefore, it is crucial to develop rapid, nondestructive technology to identify terpenoids in plant essential oils.
Molecular spectroscopic techniques such as Fourier transform infrared (FT-IR), and Raman spectroscopies have shown superior application in the handling of qualitative or quantitative researches in food and agricultural products due to their specific advantages when compare with NIR (near-infrared) spectroscopy. For example, both FT-IR and Raman spectroscopy has no influence of overtones and combination bands which are generally observed in NIR spectroscopy. Moreover, when compared with the traditional destructive chemical analysis procedures, both offer quick, simple sample preparation and non-destructive measurements. FT-IR and Raman spectroscopies have been used successfully in a number of studies to perform non-destructive evaluations of various products, such as red wines (Joshi et al., 2021), Sudan dye (Lohumi et al., 2017), Grignard reagent (Joshi et al., 2020), melamine and cyanuric acid-contaminated pet food (Joshi et al., 2023a), fabricated eggs determination (Joshi et al., 2022), and others. The identification of chemical components remains a difficult task due to the presence of various chemical compounds resulting in spectral variability. The direct observation of spectra results in the generation of incorrect information about the presence of different chemical constituents. To overcome this, it is essential to combine multivariate analysis techniques with spectrum data, thereby revealing the hidden chemical information present in the samples (Seo et al., 2021; Kim et al., 2022). For example, Huang et al. (2022) utilized Raman spectroscopy with chemometrics for the authentication and detection of adulterated agarwood essential oils. Sufriadi et al. (2021) also used principal component analysis with FT-IR spectroscopy for the discrimination of patchouli essential oils based on different geographical areas in Aceh. Also, Divyanth et al. (2022) used an application of chemometrics methods the non−destructive prediction of Nicotine Content in Tobacco. Conventional machine learning techniques such as support vector machines, principal component regression, linear discriminant analysis, have certain limitations which are responsible for slowing down their performance during model analysis. However, recently developed deep learning techniques have resolved this problem, achieving excellent performance. Due to its superior abilities in terms of feature extraction, preprocessing, and the identification of information in a single architecture without the need for manual adjustments, the convolutional neural network (CNN), a widely used deep−learning approach, has established itself in several areas of scientific research (Chatzidakis and Botton, 2019; Jung et al., 2021; Sihalath et al., 2021; Putra et al., 2022). Fuentes et al. (2023) used Raman spectroscopy and convolutional neural networks for monitoring biochemical radiation response in breast tumor xenografts. . In another study, (Wu et al., 2022) utilized a convolutional neural network with Raman spectroscopy to tackle the complex problem of identifying and quantifying honey adulteration, highlighting the model's capability in food authenticity assessment. Furthermore, (Kawamura et al., 2021) demonstrated the potential of convolutional neural networks by combining visible and near-IR spectroscopy for soil phosphorus prediction, emphasizing the versatility of deep learning across various domains. However, it is critical to assess the advantages and limitations of using deep learning and chemometrics. Deep learning methods, such as convolutional neural networks (CNNs) and recurrent neural networks (RNNs), on the other hand, excel at delivering highly accurate predictions by capturing complex patterns in spectral data, thus improving classification accuracy (Luo et al, 2022). They also automate the feature extraction procedure, saving time on human feature engineering. Furthermore, deep learning algorithms can effectively handle high-dimensional datasets prevalent in spectroscopy (Lotfollahi et al, 2019). Nonetheless, there are drawbacks to using deep learning and chemometrics. One major problem is the severe data requirements, as deep learning models normally thrive on huge labelled datasets, which can be sparse in chemometrics. Their fundamental complexity could hinder interpretability, making them less suited when understanding the reasoning behind forecasts is essential (Jindasa et al, 2021). Another issue is overfitting, which is especially problematic with little or noisy data (Lotfollahi et al, 2019). Furthermore, while deep learning excels in pattern identification, it may not provide precise insights into chemical interactions, implying that classical chemometric methods will remain relevant for in-depth research (Luo et al, 2022). To date, no work has been published that uses combination of machine and deep learning approach in conjunction with FT-IR, and Raman spectroscopy for the qualitative, and quantitative assessment of terpenoids in essential oils. The following two statements can, therefore, be used to describe the study's goals in more detail: (1) Comparative prediction analysis of terpenoids in medicinal plants essential oils utilizing partial least squares regression, support vector regression and one-dimensional convolutional neural networks. (2) Rapid classification of essential oils based on geographic locations using support vector machine classifier.
Materials and Methods
Plant Materials
In the present study, ten plant species from five families were selected. The five families were Lamiaceae and Myrtaceae, which each included a maximum number (three) of plant species; Asteraceae included two plant species, while both Rutaceae and Verbenaceae included one species each. The ten plant species were: Melaleuca linariifolia Sm (S1), Melaleuca bracteata F. Muell (S2), Callistemon citratus (S4), Murraya koeingii (S7), Lanata Camara (S10), Ageratum conyzoides (S12), Wedelia chinesis (T2), Ocimum gratissimum (O1), Ocimum kilimandascharicum (O2), and Thymus linearis (T1). Fresh plant material from the ten selected species was collected in the year 2022 from a wild region between Kathgodam (latitude 29.24◦N and longitude 79.53◦E, 554 m) and Pantnagar (latitude and longitude) from Nainital District of Uttarakhand, India. Fig. 1 below presents the images of some of the plants species which were collected during sample preparation and further utilized for essential oil extraction using chemical analysis methods.
Isolation of essential oil
Each sample was made by first cleaning the fresh aerial part of the plant, followed by a 4-hour hydro-distillation process in a device of the Clevenger type (Clevenger, 1928). This process was done to extract the essential oil (Clevenger, 1928). Flowers were hydro-distilled separately from the plant's aerial portion (its stems and leaves). Essential oil was measured directly in the extraction burette, and contents (%) were determined as the volume (mL) of essential oil per 200 g of freshly weighed plant material. After being dried over anhydrous Na2 SO4 , the crude oil was stored in a refrigerator until samples underwent GC-FID (gas chromatography-flame ionization detection) analysis.
GC-FID analysis
The 0.2 µL neat essential oils were analyzed by using GC Thermo Fischer Trace-1300 (Thermo Fisher Scientific Inc., USA). The capillary column type was TG-5MS (30 m × 0.25 mm, 0.25 µm film thickness). The carrier gas was Nitrogen at constant flow rate of 1.0 mL·min-1 and average velocity of 30 mL·min-1. The injector temperature was 240℃, the split ratio was 1 : 40, and the detector (flame ionization detector, FID) temperature was 250℃. The initial column oven temperature was set at 70℃ to 220℃ at the rate of 4℃·min-1 . The relative content of each constituent was calculated based on the % peak area (FID response) without using a correction factor. The com-position of the terpenoids found in ten different essential oils, as determined by the GC-FID reference analysis method, is shown in Table 1 below.
Table 1
The reference values of terpenoids (%) in essential oils acquired through GC-FID analysis for ten categories (S1, S2, S4, S7, S10, S12, O1, O2, T1, T2) of samples.
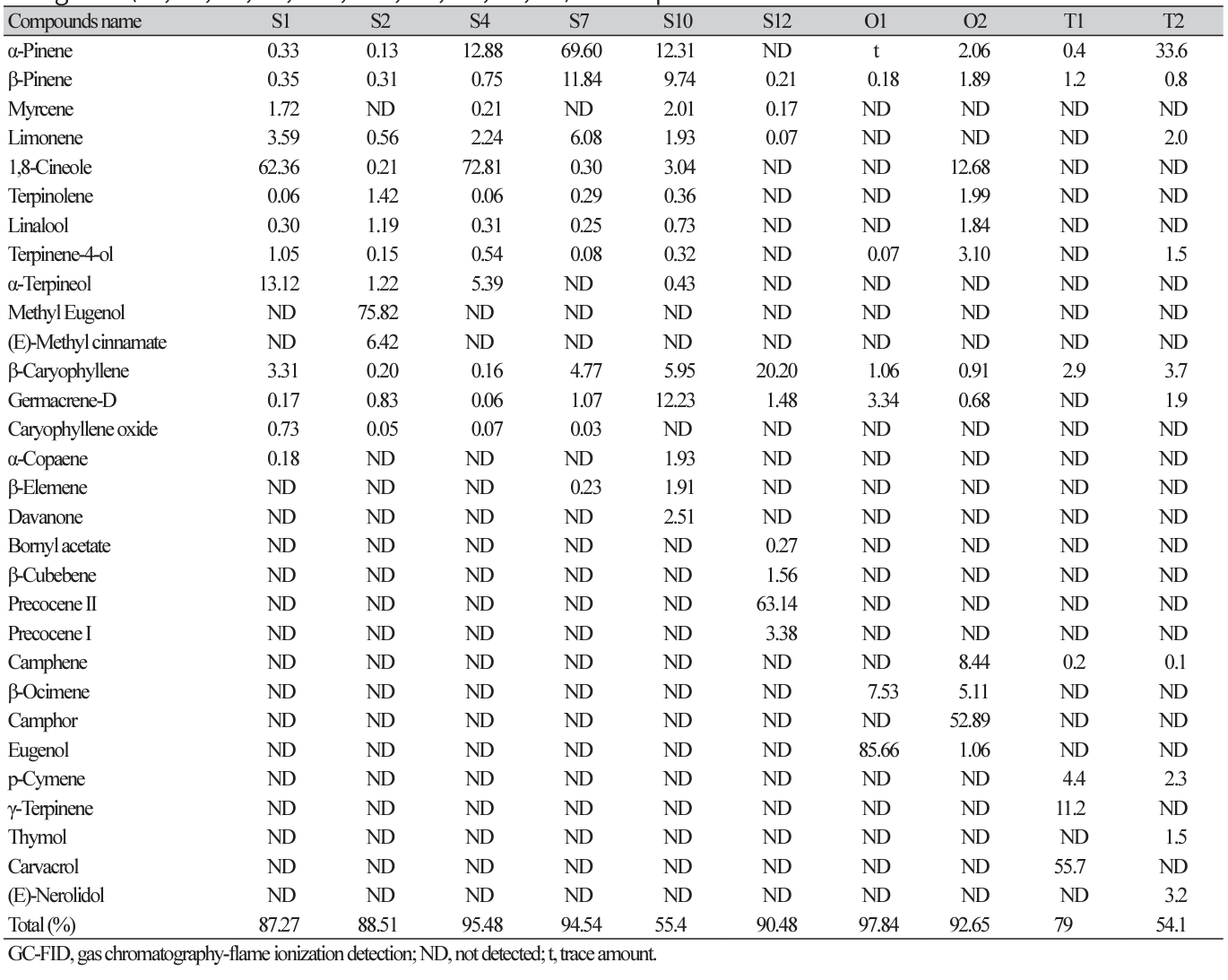
Analysis of essential oil
Identification of essential oil constituents was accomplished on the basis of retention index (RI), determined with reference to homologous series of n-alkanes, C8 - C24; Supelco Analytical, Bellefonte PA, USA, under same temperature-programmed conditions. The relative content of individual components of the oil is expressed as percent peak area relative to total peak area of the GC-FID chromatogram automated electronic integration without response factor correction.
FT-IR Spectroscopy
A Nicolet 6700 (Thermo Fisher Scientific Inc., USA) ATR−FT-IR spectrometer was used in a lab to examine the FT-IR spectrum of the essential oil samples. The attenuated total reflectance (ATR) sample mode was installed in the spectrometer. The system also utilized a deuterated triglycine sulfate (DTGS) detector and a beam splitter made of potassium bromide (KBr), both of which were managed by the OMINIC software (Thermo Fisher Scientific Inc., USA). Spectral acquisition was performed at wavelengths from 400 to 4,000 cm-1. For the spectral acquisition, each sample was placed on the surface of the diamond crystal sampling plate. Each sample was subjected to a total of 32 scans at 4 cm-1 spectral intervals, and the average spectral data were stored in Excel for-mat for future analysis.
Raman Spectroscopy
A portable i-Raman spectrometer (B&W TEK Inc., USA) outfitted with a charge-coupled de-vice (CCD) detector, and with a pixel size of 14 × 900 m and a 785-nm laser was used to record the Raman spectra of the essential oils from different geographical locations. To prevent light interference during spectral acquisition, the chemical sample Raman spectra were all acquired in a dark environment. With a laser light source operating at a wavelength of 785 nm and a power of 200 mW, spectra were obtained for each sample with an expo-sure time of 1 second and a spectral resolution of 2 cm−1. The experiment used the BAC100 model (B&W TEK Inc., USA) as a standard probe. Prior to measurement during spectral collection, the cuvette was dried. Each sample was then injected with a pipette into the cuvette from the top. The sample was then placed in front of the probe, which had been calibrated beforehand to make sure the laser would reach the sample inside the cuvette, at a distance of 2 mm. High-quality Raman spectra were produced using four scans with an integration duration of 10,000 ms, and the averaged spectra of each sample were used for model construction.
Spectral preprocessing, and chemometrics analysis
Since fluorescence background signals frequently affect Raman spectra, they contribute to masking valuable information about the chemical constitution of essential oils. The polynomial curve-fitting method was used to address this problem due to its quick processing speed and simplicity compared with other fluorescence correction methods such as wavelet transformation and Fourier transformation. The main idea behind the polynomial curve approach is to employ iterative calculations to determine the proper order of polynomials. Fig. 2a and b presents the fluorescence effected, and polynomial corrected Raman spectra. In this investigation, an 8th order polynomial with 100 iterations was selected to remove the f luorescence background.
The spectral data collected using both types of spectroscopic equipment required preprocessing procedures, essential for preventing undesirable scattering and noise effects. In this study, preprocessing processes included range normalization, multiplicative signal correction (MSC), standard normal variate (SNV), and Savitzky-Golay (SG) derivatives (first and second) to the acquired raw spectral data. Mean normalization is the preprocessing technique that is most frequently employed. Finding the mean values for each dataset is the main idea. In contrast, the maximum or range values are subtracted from each data point in the max and range normalization. On the other hand, MSC and SNV preprocessing played an important role for the elimination of background offset, slope, and scattering effect from the data. However, the overlapping peaks in the spectra were resolved and the additive effects were reduced by using the Salvitzky-Golay filters (SG-first and second derivatives) (Rinnan et al., 2009). Later, machine learning and deep learning models were created using the preprocessed FT-IR and Raman spectral data. The deep learning model was created and executed using Python, whereas the machine learning model was created using MATLAB (version 7, The MathWorks Inc., USA). Fig. 3 shows a flowchart for the FT−IR and Raman spectral analysis procedure for the samples of essential oils from different geographical locations.
Fig. 3
Diagram illustrating the full procedure explaining the workflow for FT-IR (Fourier transform infrared) and Raman spectroscopy for the terpenoids prediction in essential oils analysis. ATR-FTIR, attenuated total reflectance-Fourier transform infrared; GC-FID, gas chromatography-flame ionization detection; R2 pre, correlation coefficient for prediction; RMSEP, root mean squared error of prediction.
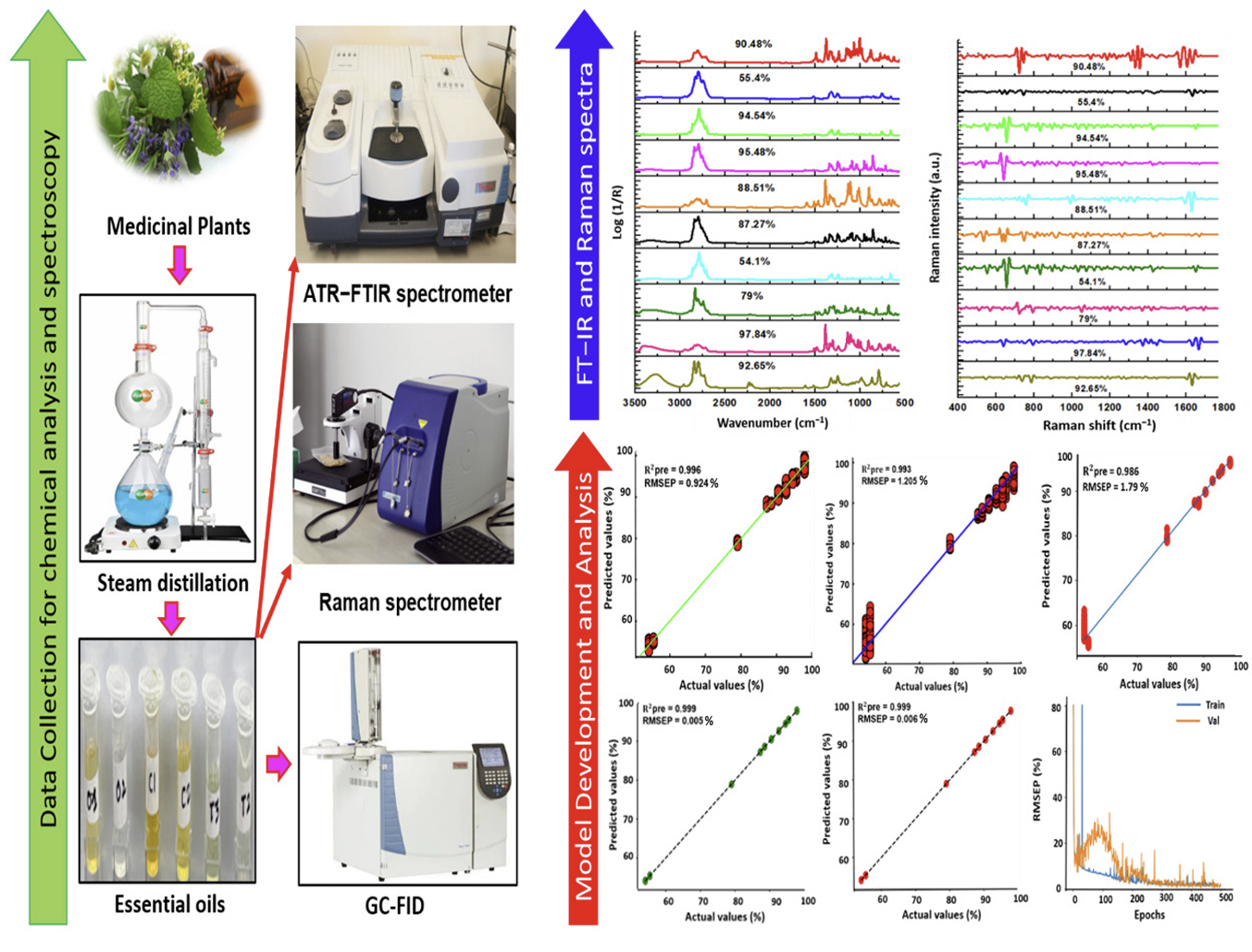
Regression and classification analysis
To perform the non−destructive quantitative evaluation of terpenoids in essential oils from different geographical locations, two widely used regression analysis methods i.e., partial least squares, and support vector machine regression were utilized in this study. Both model prediction analysis performance was compared with the GC-FID reference analysis method utilized in this research. PLSR (partial least squares regression) is very popular analytical vibrational spectroscopy methods for component quantification. It is extensively used in the spectroscopy community to relate spectral data to the physical or chemical feature being measured. In PLSR, the predictor X (predictors) and the dependent variable y (degree of adulteration) are both split into orthogonal structures referred to as latent variables (LVs), which indicate the largest correlation between X and Y (Wold et al., 2001). On the other hand, the support vector machine (SVM), a subclass of machine learning method, carries out both classification and regression tasks for the spectrum analysis. Support vectors were employed to find a hyperplane that closely corresponds to the relationship between continuous target variables and input variables. The SVM aims to increase the margin while reducing the error to a preset level (Cortes and Vapnik, 1995). SVR can manage nonlinear interactions using the kernel approach. In this work, 10 different geographic regions' worth of essential oils were collected, and we used a support vector machine (SVM) as a classification technique for the qualitative discrimination of those oils. Researchers have demonstrated the strong potential of PLSR, and SVR algorithm in the areas of plants and food products with different spectroscopic techniques like FT-IR (Elzey et al., 2016), and Raman spectroscopy (Li et al., 2021).
1D CNN model architecture
The inquiry inputs of the 1D CNN model were one-dimensional data. The features were obtained utilizing a onedimensional convolution kernel. In this study, a deep learning-based quantitative analysis model named 1D CNN was created similar to those in our previously published report (Joshi et al., 2023b) with little modifications using FT-IR, and Raman spectral data for the quantitative evaluation of terpenoids in essential oils and its performance was compared to that of machine learning regression techniques such as PLSR, and SVR. The model comprised of three convolutional layers (Conv1D_1, 2, and 3), one max-pooling layer, one flattened layer, three dense layers (Dense 1, 2, and 3), rectified linear unit (ReLU) as an activation function, and a regression output layer. Fig. 4 depicts the 1D CNN model. Fig. 4a, b presents the FT-IR, and Raman spectral data for the essential oils from different geographical locations, which consists of terpenoids of varying concentrations. Fig. 4c represents the detailed information linked to the architecture of the designed 1D CNN model for this study. The Jupyter framework and Python 3.11 with Tensor Flow were used to implement the 1D CNN models. Table 2 lists the 1D CNN architecture specifications and all the parameters used during the training process for both chemicals added to pet food.
The input layer for the 1D CNN model is made up of FT-IR and Raman spectra with an input size of 3,800 - 500 cm-1, and 400 - 1,800 cm−1 for each component of the 1D CNN. Additionally, three distinct 1D CNN layers were used for feature extraction in the 1D CNN model, each with a different layer of filters of varied sizes. To improve the performance of the developed model, alternate kernel sizes and dropout layers were selected to minimize overfitting. Additionally, the ReLU activation function was used which often follows a convolutional layer. Further, max pooling a widely employed operation in CNN architectures was implemented to down sample feature maps, expand the receptive field, and give resistance to tiny spatial translations. Max pooling with a value of 2 was used, and root mean square error and loss function were used to evaluate performance.
Results and Discussion
Raw spectra for FT-IR and Raman spectroscopies
The raw spectra recorded using FT-IR and Raman spectroscopy are displayed in Fig. 5a, b. The figure shows multiple overlapping peaks caused by a variety of undesirable background characteristics, such as instrument drift, scattering effects, noises, etc. The aforementioned effects are capable of lowering the spectral quality and thereafter reducing prediction and classification performances. To avoid these problems and obtain the meaningful data required for terpenoids prediction in essential oils, it is essential to choose the best preprocessing steps. Many pre-processing techniques, including normalization (mean and range), multiple scattering correction, standard normal variate (SNV), and Savitzky Golay derivatives (1st, and 2nd), were used in this work Based on the root mean square error levels, a single optimal preprocessing was then selected from among these processes.
Spectral interpretation for preprocessed FT-IR and Raman spectra for essential oils
The range-normalized FT-IR spectra for essential oils and the Savitzky-Golay 2nd derivative Raman spectroscopy preprocessed spectra are presented in Fig. 6a, b.
The FT-IR spectra were plotted in the 500 - 3,500 cm−1 wavenumber range, while the remaining regions i.e., below 500 cm−1, and above 3,500 cm−1, were omitted due to the lack of pertinent information required for terpenoids composition analysis. On the other hand, Raman spectra were plotted from 400 - 1,800 cm−1, respectively. The results of both spectra represent several characteristics peaks for ten different concentrations of terpenoids present in the essential oils. Since several different
terpenoids compounds were identified using the GC-FID analysis method in all the ten different categories of essential oils, highlighting their spectral peaks would be a time-consuming and difficult process. To overcome this problem, the most particular terpenoids that were present in higher concentrations were selected for spectrum interpretation and their chemical structures are presented in Fig. 7.
All the six different terpenoids identified through GC-FID reference analysis method de-liver specific spectral signatures which are clearly presented in Tables 3, and Table 4 for the FT-IR, and Raman spectral data.
Table 3
FT-IR and Raman characteristics vibrations of terpenoids in ten different categories of essential oils.
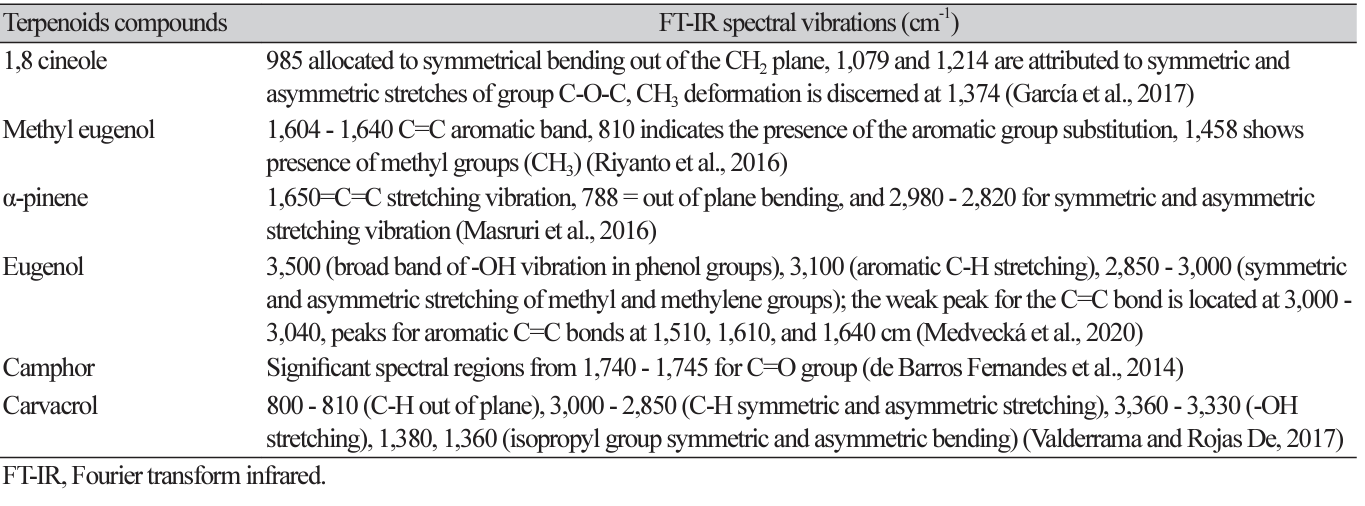
Table 4
Raman characteristics vibrations for terpenoids in ten different categories of essential oils.
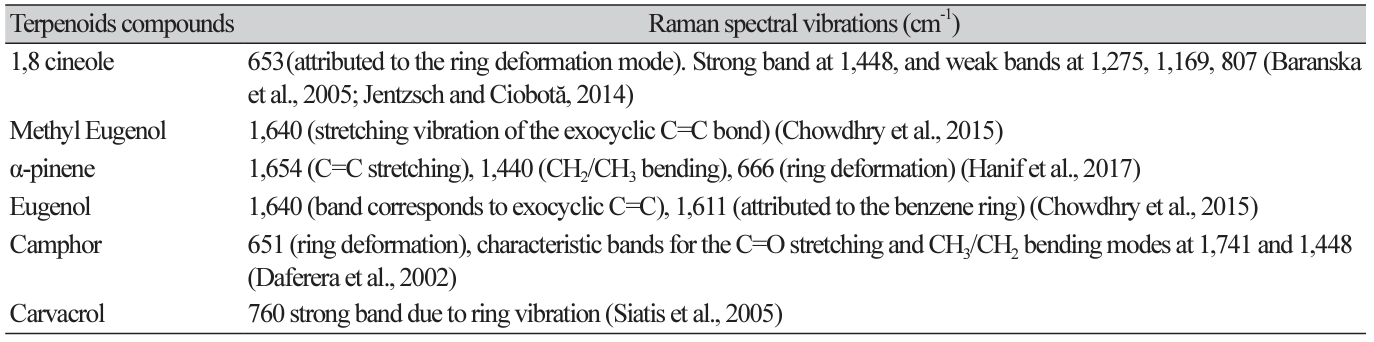
Dirichlet distribution
For the purpose of this investigation, 30 samples were collected for each of the categories of essential oils using FT-IR and Raman spectroscopy. The number of samples chosen during the model creation process had a significant impact on how effectively the model was built. In this research, a phenomenon introduced by Dirichlet was exploited to avoid the impact of underfitting during machine learning. The mathematical description of this phenomenon is thoroughly detailed (Wang et al., 2019). This approach was used to create 3,000 artificial samples prior to the development of the models, which were then used to build machine learning models for both spectroscopic systems. According to previously published researches, this algorithm exhibits strong potential for the qualitative analysis of carbaryl pesticide in food products (Joshi et al., 2023b), the presence of melamine, and cyanuric acid in pet food [15], and nitrogen in soils (Patel et al., 2020). Fig. 8a, b describes the overall concept of the Dirichlet distribution used in our work. In this context, “Original sample” refers to the spectra produced for two replicates during FT-IR and Raman data collection (i.e., 79_1 and 79_5% samples, respectively), while “Sample without noise” refers to spectra that were preprocessed as a result of the production of false data in Fig. 8b.
Fig. 8
FT-IR (Fourier transform infrared) spectra created between two replicates 1_1, and 1_2 for one category of essential oil (a). A total of 300 artificial samples were generated for FT-IR spectra for one category of essential oil using Dirichlet distribution (b). Raman spectra created between two replicates O1_1, and O_5 for one category of essential oil (c). A total of 300 artificial samples were generated for FT-IR spectra for one category of essential oil using Dirichlet distribution (d).
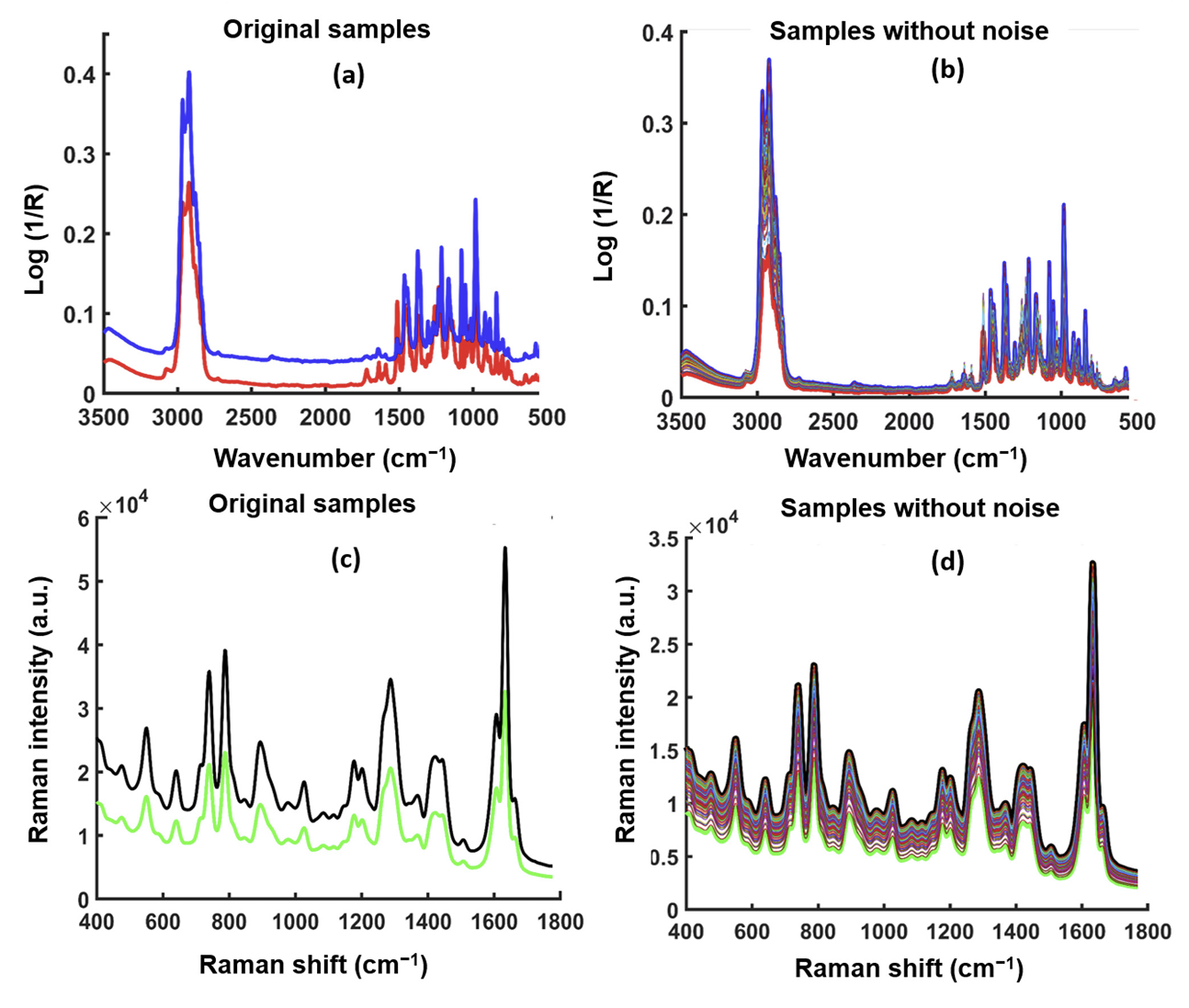
FT-IR and Raman spectroscopy model construction
The Dirichlet distribution technique produces 3,000 artificial samples that were later used to create qualitative and quantitative models. The data were splitted into calibration and prediction sets in a ratio of 70 : 30, where 2,000 samples were used as the training or calibration set and the remaining 1,000 samples were shifted to prediction or testing set. After this process, both the classification and regression models like SVC, PLSR, SVR, and 1 D CNN were developed. During the model development process, the selection of an ideal number of latent variables (LVs) is crucial to prevent overfitting issues within the model. The LVs selection was performed based on the root mean square error method during the cross−validation (leave−one out) process. Table 5 provides the complete details regarding the datasets used during model construction:
SVC classification results for FT-IR, and Raman spectroscopy
We performed a multi-class classification task on ten plant species to predict the class labels of the samples. In total, the dataset comprised of 3,000 Raman spectral observations and 3,000 FTIR spectral observation analyzed separately. Each set of data contained 2,040 spectral variables. We used a Support Vector Machine (SVM) classifier with the radial basis function (RBF) kernel and optimized the hyper parameters C and gamma through a grid search approach. The optimized hyper parameters were C = 0.0083 and gamma = scaler. The RBF kernel enabled the creation of a nonlinear decision boundary. By mapping the feature space to a higher-dimensional space, this kernel effectively captures complex relationships within the spectral data. The decision boundary created by the RBF kernel resulted in a smooth separation between the classes, allowing for accurate classification. The kernel is mathematically written as:
where l is the length scale of the kernel and d(χi , χj ) is the Euclidean distance (Duvenaud, 2014).
Fig. 9a, b shows the confusion matrices for the classification analysis results obtained by the Support Vector Machine (SVM) classifier for the FT-IR and Raman spectroscopic data. The results clearly show that the Raman spectroscopy based SVC model performed better than the FT-IR model, an acquired overall accuracy of 0.997 for the classification of essential oils from ten different geographical locations.
PLSR, and SVR analysis results for FT-IR and Raman spectroscopy
To accurately predict the composition of the terpenoids present in the essential oils, PLSR models were first developed using reference analysis findings obtained using GC-FID chemical analysis methods for the preprocessed FT-IR and Raman spectroscopy data. The models were subjected to several spectral treatments including normalization, MSC, SNV, and f irst and second SG derivatives. Of these techniques, range normalization were selected as an optimum, for FT-IR, while Savitzky-Golay 2 nd derivative was selected for Raman spectral data. With the use of seven, and eight LVS, respectively
Fig. 9
FT-IR (Fourier transform infrared) spectra created between two replicates 1_1, and 1_2 for one category of essential oil (a). A total of 300 artificial samples were generated for FT-IR spectra for one category of essential oil using Dirichlet distribution (b). Raman spectra created between two replicates O1_1, and O_5 for one category of essential oil (c). A total of 300 artificial samples were generated for FT-IR spectra for one category of essential oil using Dirichlet distribution (d).
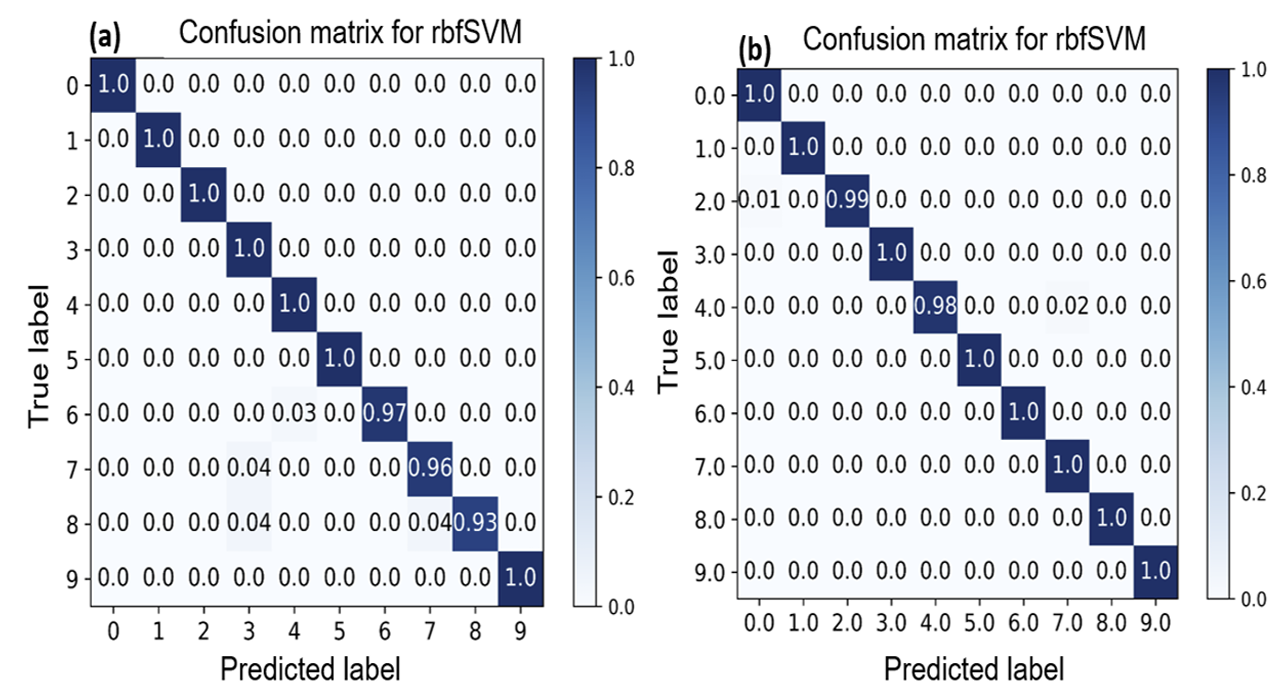
both PLSR models produced prediction correlation coefficients (R2) of 0.996 and 0.993 together with RMSEP values of 0.924% and 1.205%. For FT-IR and Raman data, Fig. 10a, b shows a prediction analysis plot created by the PLSR model, demonstrating a significant correlation between actual and predicted values and showing that the model was appropriate for the quantitative analysis of terpenoids in essential oils.
Another widely used regression model SVR was created for both FT-IR and Raman spectral data to compare its prediction performances with the previously developed PLSR model. During SVR model construction, choosing an optimum kernel is very important. In this study, two different routinely used kernels namely linear, and radial basis function (rbf), were utilized. Of these two kernels, the linear kernel acquired the highest prediction performance for both spectroscopic techniques and resulted in the highest R2 of 0.999 with the lowest RMSEP value of 0.005%, and 0.006%. The overall prediction analysis f indings for both machine learning models are displayed in Table 6 below. Fig. 10c, d presented above also displays the prediction analysis plots created using the SVR models for the FT-IR and Raman datasets, which illustrate the linear correlations created between actual and predicted values. According to the results, shown in Table 6, it is abundantly obvious that the SVR models based on FT-IR spectroscopy outperformed the above developed PLSR models and achieved lower RMSE values during the quantitative analysis of terpene compositions in plant essential oils.
Fig. 10
PLSR (partial least squares regression) and SVR (support vector regression) prediction analysis plot between actual and predicted concentrations of terpenoids in essential oils for (a, c) FT-IR (Fourier transform infrared), and (b, d) Raman spectroscopy. R2 pre, correlation coefficient for prediction; RMSEP, root mean squared error of prediction.
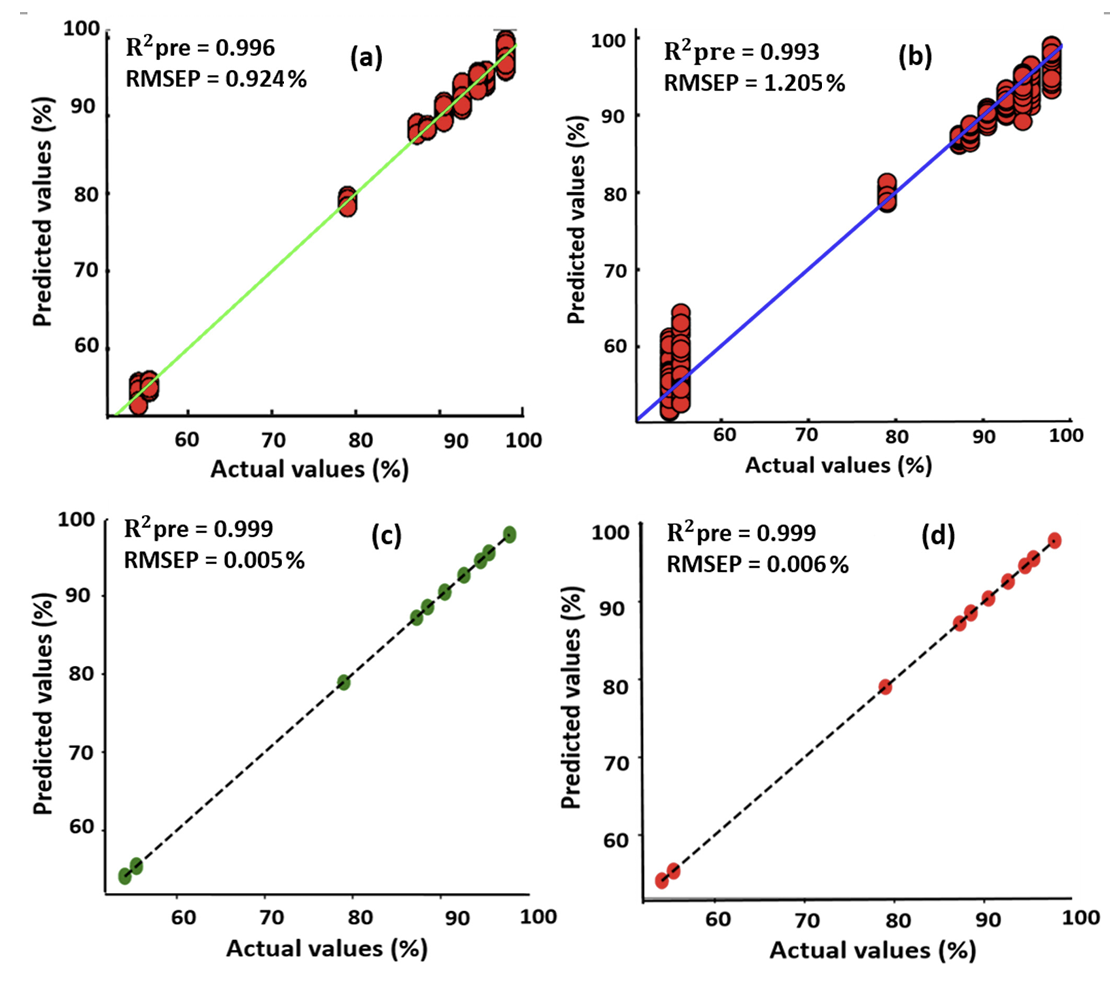
1 D CNN analysis results for FT-IR and Raman spectroscopy
In this study, a deep learning-based model named 1 D CNN was created in order to assess how well it performed in comparison to the machine learning models created in Section 3.6 for the quantitative prediction of terpenoids in essential oils. The model's full design is presented in depth in Section 2.9. Equal numbers of plant essential oil samples were employed in the creation of the 1D CNN model, and the correlation coefficient (R2) and RMSEP were utilized as well as performance evaluation indicators. A total of 3,000 samples that resembled machine learning models were employed; 2,000 of these samples were moved to the calibration set, and the remaining samples were used for the prediction dataset for both FT-IR and Raman spectroscopic data. The model comprises three separate convolution layers with a dropout value of 0.5 make up the model. The ReLU activation function was chosen, and the learning rate for 1D CNN models was 0.001. The values of the epochs were changed and the best 500 epochs during the training process were selected; a total of 1,053,745 training parameters were used. The parameters were carefully adjusted in order to maximize prediction performance. The prediction analysis results and loss−function curve obtained using the developed 1 D CNN model for the FT-IR spectroscopic data are shown in Fig. 11a, b, respectively. The developed deep learning model resulted in a R2 value of 0.999 and with an RMSEP value of 1.10 % which is comparatively higher than those of previously constructed machine learning models. Further, the resulting loss−function curve be attaining convergence at 400 epochs for the training and validation datasets.
Similarly, another 1D CNN model was constructed for the acquired Raman spectral data using a similar number of samples for use the during training and validation process. The prediction analysis results and loss function curve obtained using the developed 1D CNN model for Raman spectroscopic data are shown in Fig. 11c, d above respectively. The developed 1D CNN model yielded a higher correlation coefficient (R2) value of 0.986 with the lowest RMSEP value of 1.79 %. Further, for the training and validation datasets, convergence began after 400, which is shown in loss−function curve.
Fig. 11
1D CNN (one-dimensional convolutional neural network) models prediction analysis results for terpenoids prediction in essential oils for (a, b) FT-IR (Fourier transform infrared), and (c, d) Raman spectroscopy. Here the figures (b, d) are loss function curves. R2 pre, correlation coefficient for prediction; RMSEP, root mean squared error of prediction.
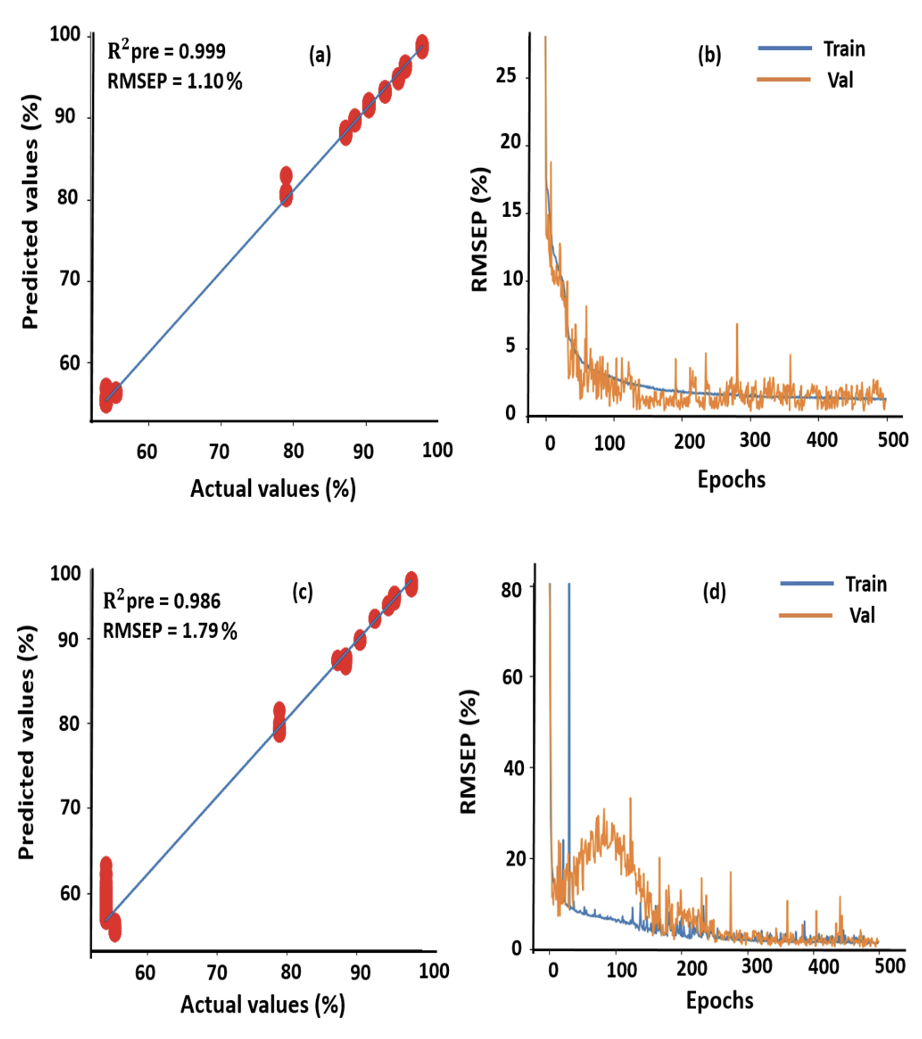
The two bar charts shown in Fig. 12a, b display the overall performance assessment results of the machine learning (PLSR, SVR) and 1 D CNN models for FT-IR and Raman spectroscopic data. The RMSEP values and correlation coefficient (R2) variations for the prediction datasets are shown in both bar charts. In terms of R2 and RMSEP values, the bar charts unambiguously demonstrate that support vector regression models outperformed both PLSR and 1D CNN models. Table 7 displays the outcomes of the prediction analysis for each of the three regression analysis models that were used on the prediction dataset produced by this study.
Fig. 12
Performance assessment of the PLSR (partial least squares regression), SVR (support vector regression), and 1D CNN (one-dimensional convolutional neural network) machine and deep learning models in terms of correlation coefficient and prediction error for (a) FT-IR (Fourier transform infrared), and (b) Raman spectroscopic data using bar−chart figures. R2, correlation coefficient for prediction; RMSEP, root mean squared error of prediction.
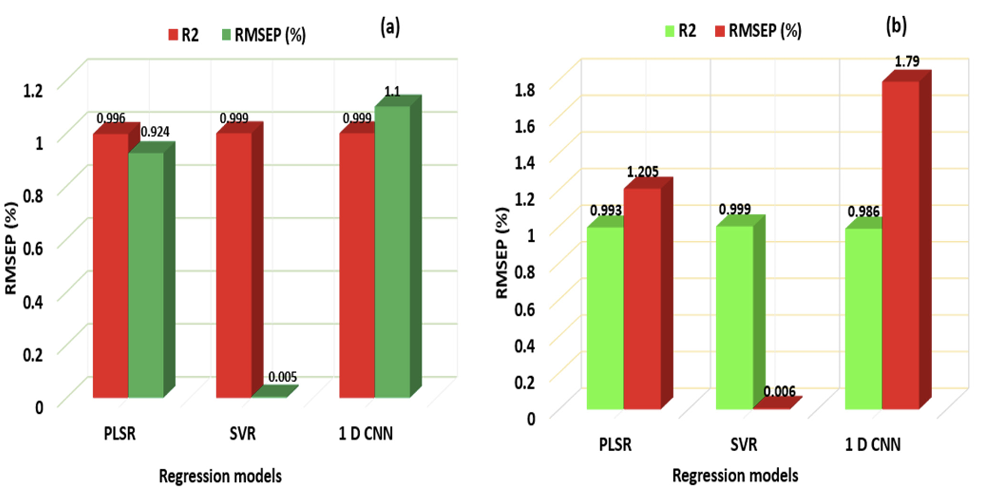
The acquired findings presented in Table 7, confirm the performance of the SVR model. Hence, it could be clearly suggested that FT-IR spectroscopy, when combined with the support vector regression model is very effective for the nondestructive examination of terpenoids in essential oils, requiring less sample preparation and achieving faster evaluation. In earlier research, Rodríguez-Maecker et al. (2017) used a static headspace gas chromatography-ion mobility spectrometry method to identify terpenes with an R2 correlation coefficient of 0.999. The measurement of terpenes in essential oils obtained from conifers was also carried out by Allenspach et al. (2020) utilizing gas chromatography in conjunction with a flame ionization detector with a higher LOD, yielding a strong R2 value of 0.999. Due to their sophisticated sample preparation processes, the practical use of these approaches in the real world is laborious and time-consuming. However, because this study analyses more samples while concurrently creating machine learning and deep learning models, the sample preparation process is quick and easy. Consequently, the results unambiguously demonstrate that support vector regression (SVR) in combination with ATR-based FT-IR spectroscopy is particularly capable of the quick and nondestructive evaluation of terpenoids in various kinds of medicinal plants essential oils. Because the spectrum data includes multiple variables that are nonetheless highly significant and connected to functional groups found in chemical entities. However, the inclusion of these variables causes the multivariate analysis computing time to increase. Future works will expand on this research by using band selection techniques like variable importance in projection (VIP), successive projection algorithm (SPA), and genetic algorithms (GA) to remove undesirable variables and enhance performance by speeding up data processing. Additionally, additional samples of plant essential oils in various combinations with other phytochemicals will be examined to see how well our established method works for non-destructive quantitative evaluation.
Conclusion
In this study, two different vibrational spectroscopy methods�ATR−FT-IR and Raman spectroscopy were used to evaluate terpenes composition in medicinal plant essential oils for comparison against gas chromatography coupled with a f lame ionization detector (GC-FID), a standard analysis technique. Both spectroscopic methods produced interesting findings, however FT-IR spectroscopy outperformed Raman spectroscopy. The non-destructive quantitative prediction of terpenoids, was performed using PLSR, SVR, and 1D CNN models. The SVR model outperformed other models using FT-IR spectroscopy and acquired the highest correlation coefficient of 0.999 with an RMSEP error of 0.006%, which is somewhat better than the Raman data. On the other hand, the qualitative separation of essential oils from ten distinct geographic locations was conducted using the support vector classification method, which obtained the greatest accuracy of 0.986 and 0.997% utilizing FT-IR and Raman spectroscopic data. The acquired R2 and RMSEP values demonstrate the potential of our developed model. Based on these results, it can be concluded that ATR-FT-IR spectroscopy in conjunction with the SVR machine learning technique offers a strong capability for the rapid, exact, and nondestructive identification of terpenoids presents in different varieties of essential oils. In contrast to more established destructive techniques such as HPLC, and GC-MS, which have low detection limits, the developed method shows promise for the quick, chemical-free, and effective detection of product adulteration because it does not require a sophisticated lab or trained personnel to conduct analyses.
Acknowledgements
This work was supported by Korea Institute of Planning and Evaluation for Technology in Food, Agriculture and Forestry (IPET) through Smart Farm Innovation Technology Development Program, funded by Ministry of Agriculture, Food and Rural Affairs (MAFRA) (421005-04). Further, we also give deep thanks for Department of Chemistry, M.B.G.P.G College Haldwani, Kumaun University Nainital-263139, Uttarakhand, India for their collaboration during plants sample collection and their essential oils extraction and later terpenoids determination using GC-FID chemical analysis methods.
Authors Information
Rahul Joshi, https://orcid.org/0000-0002-5834-2893
Sushma Kholiya, https://orcid.org/0009-0007-4066-0539
Himanshu Pandey, https://orcid.org/0000-0001-5419-8903
Ritu Joshi, Texas A&M University
Omia Emmanuel, https://orcid.org/0000-0001-8105-3304
Ameeta Tewari, https://orcid.org/0009-0007-5571-5635
Taehyun Kim, National Institute of Agricultural Science, Rural Development Administration
Byoung-Kwan Cho, https://orcid.org/0000-0002-8397-9853