Introduction
Mycotoxins are toxic secondary metabolites produced by harmful fungi, which cause diseases, and exert certain physiological effects on humans and livestock (Peraica et al., 1999; Kim et al., 2017). In particular, Fusarium mycotoxins produced by different Fusarium species are commonly observed in food and feedstuff (Zain, 2011). Contamination of food and feed with mycotoxins is a serious health problem in several countries worldwide. Fusarium mycotoxins constitute the root cause of substantial economic losses to the agricultural industry, because of long-term exposure to dietary ingredients. Pigs are relatively more sensitive to mycotoxins than other organisms (Suleiman et al., 2013).
Among mycotoxins, deoxynivalenol (DON) reduced feed intake in pigs, while ruminants and poultry were observed to be less sensitive to mycotoxins (Murugesan et al., 2015). The representative trichothecene mycotoxin, DON, causes vomiting, diarrhea, and weight loss in humans and animals by affecting the immune system (Anukul et al., 2013). DON is the most common contaminant of feed. Swine are more sensitive to DON than other organisms (Pinotti et al., 2016). The effects of DON on animals through multiple complex mechanisms regulate various developmental stages in organization.
Metabolomics is the large-scale assessment of biological systems with the strongest correlation to phenotype or environmental processes (Krumsiek et al., 2016; Misra et al., 2017). Metabolomic studies are required to enhance the understanding of biological systems contaminated with mycotoxins. Proton nuclear magnetic resonance (1H NMR) spectroscopy together with multivariate statistical analysis is established as an effective method for screening metabolic responses. Therefore, it is interesting to determine the toxic effects of mycotoxins present in animal feed. This study was aimed at investigating the molecular effects of mycotoxins, and identifying metabolome-related compounds untargeted for DON-treated piglets.
Materials and Methods
Experimental design of treatment of piglets with DON
All procedures and experiments were approved by the Institutional Animal Use and Care Committee of the National Institute of Animal Science, Korea (No. 2015-147). From a local farm, 9 castrated male piglets (6-week-old, Landrace/Yorkshire/Duroc) of approximately equal weights (19 ± 1 kg) were obtained. Piglet was housed in a separate pen (2100 cm × 1400 cm). The piglets were acclimated at 25 ± 1°C for one week. The piglets were assigned to control (n = 4) and DON treatment groups (n = 5). Control piglets were fed with standard feed to meet the nutritional requirements, while piglets of the DON treatment group were fed with the mixture of standard feed and DON. Commercial DON was mixed with the diet at 8 mg/kg in feed using purified toxins (BIOMIN Korea Ltd., Seoul, Korea). During the experimental period (4 weeks), feed and water were provided ad libitum. The analysis of DON components was conducted using ultra performance liquid chromatography as described previously (Reddy et al., 2018).
Sample preparation and analysis
After DON treatment, the control and treated piglets were sacrificed after anesthetizing them with barbiturates and pentobarbital. Serum, liver, and urine samples, to monitor cardiac arrest, were collected immediately, frozen with liquid nitrogen, and stored at - 80°C for metabolomic analysis. Then, 45 µL of serum and urine samples, each, was mixed with 5 µL of D2O (20 mM TSP-d4). All samples from individual piglets were used for 1H-NMR metabolic profiling. Liver samples were ground to a fine powder using a mortar and pestle. Each sample was transferred to a 4 mm NMR nanotube for NMR analysis. 1H-NMR spectra were obtained by high-resolution magic-angle-spinning (HR-MAS) NMR spectroscopy using an Agilent 600 MHz NMR spectrometer (Agilent Technologies, Palo Alto, CA, USA) with a 4 mm gHX NanoProbe. A Carr-Purcell-Meiboom-Gill (CPMG) pulse sequence was used to reduce the signals generated by water and macromolecules. The 1H-NMR spectra were measured using 90° pulse for 7.25 µs , big tau of 0.15 s , relaxation delay of 3 s, acquisition time of 1.704 s, and total acquisition time of 10 min 31 s. For each sample, 128 transients were acquired. The TSP-d4 peak at 0.0 ppm was used as the reference to calibrate chemical shifts. 1H-NMR spectrum assignment and metabolite quantification were performed using Chenomx NMR Suite 7.1. Metabolite set enrichment analysis (MSEA) used a web-based server with MetaboAnalyst 4.0 (http://www.metaboanalyst.ca).
Statistical analyses
For multivariate statistical analyses, each 1H-NMR spectrum was binned with a binning size of 0.001 ppm, and normalized to the total area. The result of binning was aligned using the icoshift algorithm of MATLAB R2013b (MathWorks, Natick, MA, USA). Principal component analysis (PCA), orthogonal partial least squares discriminant analysis (OPLS-DA), and variable importance in the projection (VIP) method were performed using SIMCA-P+ (Umetrics, Umea, Sweden). Variables with VIP value exceeding 1.0 were considered important for discrimination among groups.
Results and Discussion
Multivariate analysis of liver, serum, and urine metabolites
For metabolites identification, NMR-based metabolites were assigned to spectral peaks for the serum, liver, and urine samples from the control and DON-treated piglets. PCA scores and loading plots were analyzed within the 95% Hotelling T2 ellipse (Fig. 1). PCA score plots were developed for the three different sample types, including liver, serum, and urine for 4 weeks of treatment. The PCA model characteristics were excellent: R2X = 0.827; Q2 = 0.7. The results showed that using one-dimensional (1D) NMR spectra, totally, 28 (serum), 29 (liver), and 20 (urine) metabolites were assigned to the metabolic profile. The three sample sets did not show clear differences in the unsupervised PCA classification (Fig. 1A). However, the DON-treated serum group showed obvious and significant differences from the liver and urine groups. Metabolic profiling by NMR spectroscopy revealed significantly diminished or elevated levels of potential metabolites, including lactate, glucose, taurine, alanine, glycine, glutamate, creatine, and glutamine, upon mycotoxin exposure (Fig. 1B). The VIP values of these metabolites were set at > 1.0 (Fig. 1C). The liver is an important organ for metabolism, involved in glycogenolysis and detoxification, and is important for mycotoxin metabolism (Ji et al., 2017). In this study, the metabolic profile of piglet urine revealed the effect of mycotoxin mixtures. The harmful effects of mycotoxin were suggested to be due to interactions between liver and serum metabolites in mice (Sun et al., 2014).
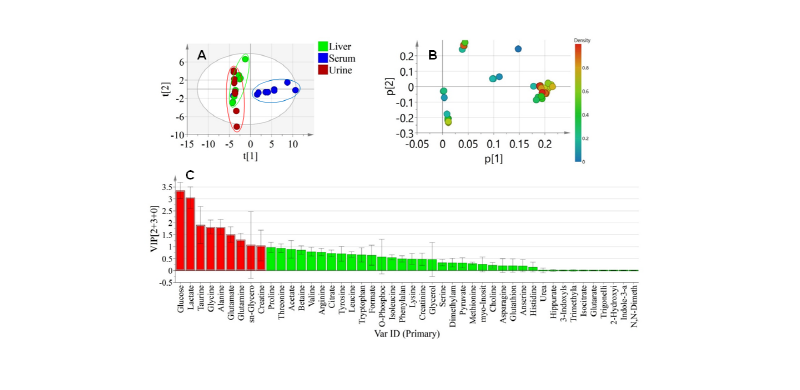
Fig. 1. Principal component analysis (PCA) of liver, serum, and urine of control (4 samples) and treated (5 samples) piglets. (A) Score plot. (B) Loading plot. R2X = 0.827; Q2 = 0.7. (C) The variable importance in projection (VIP) plots from OPLD-DA showed that significant metabolites contributed to cluster separation in piglets. Six-week-old piglets were treated with 8 mg/kg DON for 28 days. Signals were compared after log transformation and Pareto scaling.
Overall, this approach was aimed at assessing potential differences in the metabolic response of piglets fed with feed contaminated by a single mycotoxin. The exposure of immature gilts to contaminated diet showed that the pathways involved in cell proliferation and survival were disrupted (Brzuzan et al., 2015). The mycotoxins and secondary metabolites were dose-dependently quantified in vivo in swine urine (Tiemann and Danicke, 2007). DON is also the cause for cytotoxicity to fibroblasts, reticulocytes, and lymphocytes (Rotter et al., 1993). At high concentrations, it causes leukocyte apoptosis, together with immunosuppression (Pestka, 2008).
Metabolite pathway enrichment analysis
Unlike gene set enrichment analysis (GSEA), MSEA in this study, was performed to assess the patterns of changes in various metabolic pathways for predicting important metabolic pathways. MSEA was performed using MetaboAnalyst 4.0 for 77 selected metabolites based on their VIP scores. In this study, we consider the MSEA approach a powerful analytical technique to develop metabolite correlations with visualization and integrated discovery. To investigate the association between the metabolites, we performed MSEA using the web server MetaboAnalyst. Of the 46 metabolites identified, two metabolites pathway were observed to be significant (p < 0.05, false discovery rate (FDR) < 0.01), including glyceraldehyde-3-phosphate dehydrogenase and citrate synthase (Fig. 2). Finally, we suggested that glyceraldehyde-3-phosphate dehydrogenase and citrate synthase were affected by DON, because of reduction in glucose uptake. Differential metabolites revealed the partial association of the metabolites with blood pressure (Aa et al., 2010). Glutamic acid and glycine may protect against food toxicity (Zeb and Rahman, 2017). In a previous study on feed safety management of total mixed ration, contaminant levels in feed were measured (Ahn et al., 2017). Several mycotoxins, such as aflatoxins, ochratoxin A, fumonisins, zearalenone, and deoxynivalenol, in different cereals were analyzed using liquid chromatography-mass spectrometry (LC-MS) (Ok et al., 2007; Kim et al., 2013). Climate change also influences mycotoxin production (Kim and Chun, 2009).
Conclusion
These results may improve our understanding of diet-related discriminating metabolites in piglets provided with DON-treated feed. NMR partly revealed the metabolism in piglets and distinct metabolic profiles among different tissue samples. The liver metabolites were similar to those in urine. However, multivariate analysis showed that the serum metabolites were obviously distinct from the urine and liver metabolites. The integrated metabolic pathways suggested that the DON-treated diets induced differences in metabolism in piglets.
Acknowledgements
This work was conducted with the support of the Cooperative Research Program for Agriculture Science and Technology Development (Project No. PJ01093202) and the 2018 RDA fellowship program of the National Institute of Animal Sciences, Rural Development Administration, Republic of Korea.