Introduction
쌀(Oryza sativa)은 한국을 비롯한 아시아 지역의 주식으로서 주요한 곡물 중 하나이다. 국내에서는 단립종 벼를 주로 생산하는데 아밀로스 성분이 비교적 적어 차지고 윤기가 흐르는 특징이 있다. 도정 후 밥, 떡, 술 등으로 가공하여 취식하고 있으며, 최근에는 빵, 과자, 케이크 등으로 다양한 가공을 거친 후 제품화되고 있다(Bao and Bergman, 2004). 최근 환경문제, 가공비 절감, 가공성 향상 등의 목적으로 쌀가루 제조 방식에 관한 다양한 연구가 수행되고 있다. 물에 침지하여 분쇄하는 습식가공이 주를 이루던 기존의 방식은 높은 수분 함량(35%)으로 저장 및 유통과정에서 냉동 보관하는 어려움이 발생한다. 반면, 수분 함량이 12 - 14% 내외의 8시간 수침처리한 건식제분 쌀가루는 습식에 비해 저장이 용이하고, 제분 방식과 보관 방식에 따라 품질 변할 수 있는 습식에 비해 가공품질이 안정적이라는 특징을 보였다고 발표했다(Kim and Shin, 2007). 쌀의 품종별로 아밀로스 함량을 비교하고 취반 특성을 비교한 결과(Yoon et al., 2012), 건식 쌀가루를 첨가한 반건면의 품질특성에 관한 연구(Park et al., 2020) 등이 발표되었다. 쌀에 다양한 첨가물을 활용한 가공식품에 관한 연구도 지속적으로 수행되고 있다. 양잠 산물을 활용한 죽 제조(Kim et al., 2007), 버섯균사체를 활용한 쌀 가공 식품의 품질특성에 관한 연구(Kim and Chun, 2017) 등이 발표된 바 있다.
비파괴 품질검사법을 활용하여 쌀의 품질 평가를 한 연구가 꾸준히 수행되었다. 가시 및 근적외 분광법을 이용한 쌀의 정백수율(Kim et al., 1997)을 측정하거나, 도정비율(Lee and Lee, 1999)을 예측하는 연구가 수행되었으며, 곡물의 품질을 결정하는 주요 분자들인 수분, 단백질, 아미노산 등의 비파괴 측정을 위하여 빠르고, 시료의 전처리를 최소화할 수 있어 비용절감에 도움이 되며, 모델개발이 용이한 근적외 분광분석법이 사용되었다(Delwiche et al., 1995; Sohn et al., 2004; Bagchi et al., 2016; Sampaio et al., 2018; Lin et al., 2019; Rahman et al., 2019). 기계시각을 이용한 현미의 개체별 완전립, 동할립, 미탈부립 등의 품위를 측정하는 알고리즘 개발(Noh et al., 1997) 및 칼라영상처리를 이용한 현미 품위측정 시스템 연구(Kim and Noh, 2010) 등이 발표되었다. 최근에는 가시 및 근적외선 파장 대역의 초분광 영상장치를 활용하여 백미의 지형적 원산지 구분을 예측하는 모델개발에 관한 연구도 발표된 바가 있다(Mo et al., 2017). 초분광 영상기술은 1985년 초분광 영상(hyperspectral imaging)으로 처음 소개되었으며, 원격탐사분야에서 개발되어 토지정보를 관측하고 분석하는데 이용된 기술이다(Goetz, 2009). 농식품 분야에서는 2000년 이후부터 라인스캔방식의 초분광 영상장치를 이용하여 반사와 형광분광을 측정한 것을 시작으로 다양한 연구에 적용되었다(Kim et al., 2001). 초분광 영상기술은 영상정보와 분광정보를 동시에 획득할 수 있으며, 하이퍼큐브로 불리는 3차원 데이터를 활용하여 영상분석 및 분광분석이 가능하다. 최근에는 쌀 품질 판별에 초분광 영상기술(400 - 1,000 nm)을 이용하여 변색이나 유병종자를 판별한 연구 결과를 발표하기도 하였다(Baek et al., 2019).
본 연구의 목적은 라이스페이퍼 등 가공 제품에 주로 사용되는 곡물가루를 판별하기 위해 가시근적외 파장대역의 초분광 영상시스템(420 - 730 nm)을 이용하여 시료의 초분광 영상을 촬영하고 추출한 스펙트럼을 이용하여 각 시료별 판별가능성을 확인하는데 있다. 또한, 판별모델을 최적화하기 위한 전처리 알고리즘을 비교하여 판별모델의 성능향상과 산업현장에 적용가능성을 확인하고자 하였다. 이를 위하여 선형 및 비선형 다변량 분석 및 머신러닝을 이용하여 개발한 판별모델 정확도를 판별분류표(confusion matrix)를 통하여 비교하였다.
Materials and Methods
곡물 재료
총 7 품종의 가공용 쌀(미면, 박력분, 강력분)과 전분(타피오카, 묵, 감자) 등을 판별시료로 선택하여 측정하였다. 시료 중 한가루, 미면, 신길은 국립식량과학원(Wanju, Korea)에서 지원을 받았으며, 그 외 쌀가루 및 전분은 시중에서 구매하였다. 플라스틱으로 제작한 5 (L) × 5 (W) × 1.5 (H) cm3 크기의 사각통에 담아 3개씩 제작하여 측정하였다. 시료통에 담긴 시료를 구분하기 위하여 시료별 칼라 영상을 획득하였으며, 시료통 순서와 시료 명칭을 약어로 작성하였다(Fig. 2). 시료통 순서에서 C1과 C2는 첫 번째 행과 두 번째 행(column)을 의미하며, R1 - R4는 열(row)을 의미한다. 쌀 시료의 경우 약어에 F를, 전분가루의 경우 S를 붙여 분리하였다(SF [strong flour]: 강력분, MF [mimyeon flour]: 미면, WF [weak flour]: 박력분, TS [tapioca starch]: 타피오카전분, SS [sweatpotato starch]: 고구마전분, CS [chestnut starch]: 밤전분, MS [mungbean starch]: 녹두전분). 시료 중 미면은 국립농업과학원에서 두 종류의 입자 크기(MF1: 128.3 ± 0.61 μm, MF2: 76.33 ± 1.02 μm)로 직접 분쇄하여 판별용 시료로 사용하였다.
초분광 영상시스템
제작된 시료의 초분광 영상은 국립농업과학원(Jeonju, Korea)에서 데이터 획득용으로 제작한 초분광 영상측정장치를 사용하였다. 초분광 영상장치의 형광영상 획득을 위하여 자체 제작한 UV-A (365 nm) 파장의 LED 조명시스템을 사용하였으며, 선형모터(XSlide, Velmax, Bloomfield, NY, USA)로 구동되는 시료대, C-마운트 렌즈(Schneider Optics, Van Nuys, CA, USA)를 장착한 라인스캔 카메라(MegaLuca, Andor Technology, Belfast, Northern Ireland) 및 420 - 730 nm의 파장대역으로 65개의 파장을 획득하는 분광기(VNIR Hyperspec, Headwall Photonics, Fitchburg, MA, USA)를 통하여 이미지 크기 310 × 502 화소의 영상을 획득한다.
초분광 영상 보정 및 관심영역 추출
초분광 영상장치로 초분광 형광 하이퍼데이터를 획득한 후 노이즈 제거를 위하여 데이터 보정을 수행하였다(Fig. 1). 영상측정 시 물리적, 환경적 요인의 차이로 인해 발생하는 영상 노이즈를 제거하기 위하여 암흑영상(dark reference)은 외부광원을 차단하기 위하여 카메라 마개로 막은 후 측정하였다. 영상보정을 위하여 측정한 원영상(raw data)에서 암흑영상(dark reference)를 차감하여 사용하였다. 보정된 영상데이터에서 배경을 제거하고 관심영역(ROI, region of interest)을 설정한다. 영상을 이진화하는 알고리즘은 Otsu, entropy, min, max, histogram 등 다양한 방식이 사용되고 있다. 본 연구에서는 상기 알고리즘 중에서 가장 널리 사용되는 히스토그램 방식을 이용하여 배경을 제거하였다. 획득한 초분광 영상에서 10번째 영상(465.4 nm)의 히스토그램을 구한 후 최적 문턱치(TH, threshold)를 이용하여 ROI를 설정하였다.
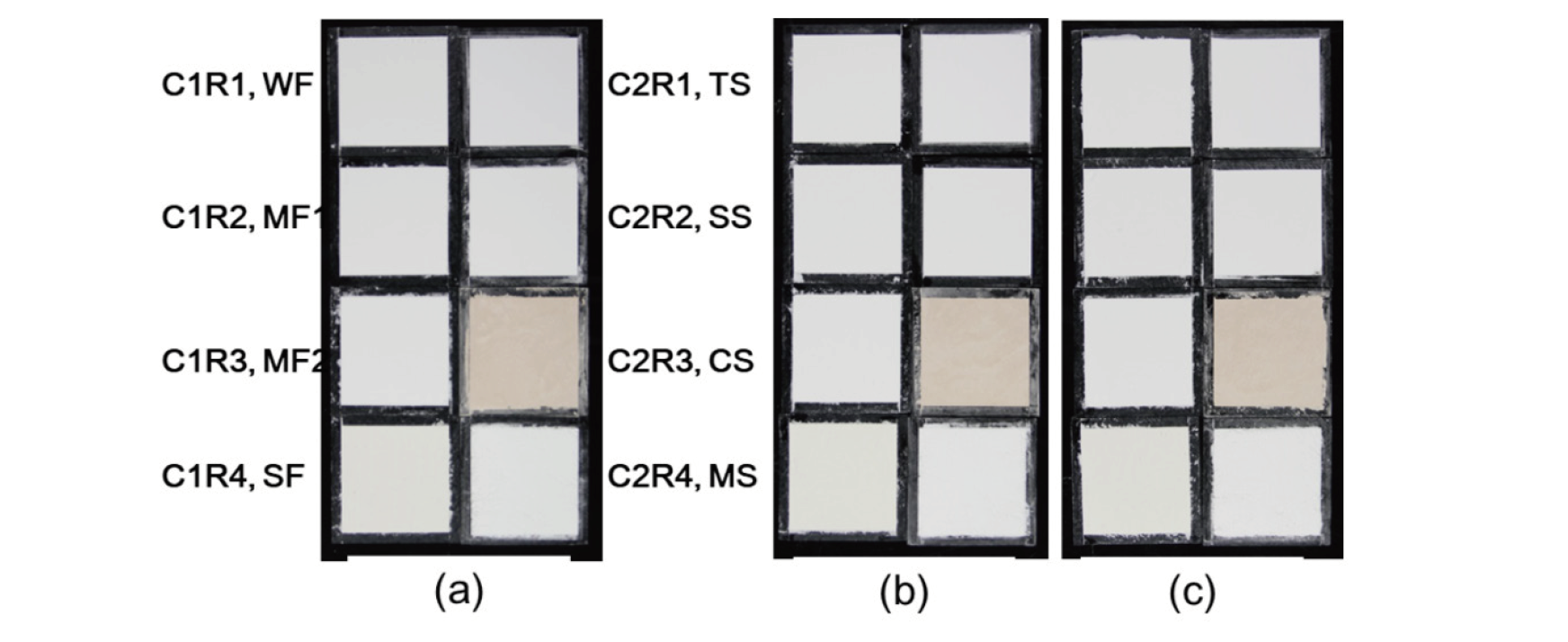
Fig. 2. Rice and starch flour samples mosaic (a) and replica (b and c). Left columns from each sample image represent rice flours and right columns are starch samples. C1 means first column and R1 means first row. C1R1, weak rice flour (WF); C1R2, mimyeon flour (MF1); C1R3, mimyeon flour (MF2); C1R4, strong rice flour (SF); C2R1, tapioca starch (TS); C2R2, sweatpotato starch (SS); C2R3, chestnut starch (CS); C2R4, mungbean starch (MS).
판별 모델 개발
획득한 스펙트럼은 특성 파악을 위하여 주성분분석(PCA, principal component analysis) 방법을 이용하여 분석을 수행하였다. 주성분분석은 대표적인 데이터 차원 축소 알고리즘으로서, 입력된 데이터들의 상관관계나 공상관 관계를 이용하여 n차원의 데이터를 2차원 혹은 3차원 주성분 공간으로 사영하여 데이터의 분산이 가장 큰 방향으로 새로운 축을 결정하게 된다. 입력 데이터들의 분산이 가장 큰 방향에 위치한 축을 1번 주성분(PC1)으로 설정하고, 그 다음 분산이 크면서 수직한 축을 2번 주성분(PC2) 등으로 설정한다. 이를 통하여 모든 데이터의 차원을 축소하거나 원점을 중심으로 데이터 군집간 상대적 거리를 확인할 수 있다(Wold et al., 1987).
라인스캔 초분광 영상장치를 이용하여 3차원 하이퍼 데이터를 획득할 때 다양한 환경적, 물리적 잡음이 포함될 수 있다. 신호 전처리 알고리즘을 이용하여 획득한 스펙트럼에서 잡음을 제거할 수 있는데 본 연구에서는 다분산보정(MSC, multiplicative scattering correction), 1차, 2차 미분(1st and 2nd derivative using Savitzky-Golay algorithm), 이동평균법(MA, moving average) 등을 적용하였다(Yang et al., 2011). MSC는 분광정보의 이상치를 설정하고 이상치와 실측치의 거리를 계산하여 산란을 보정하는 방식인데 이상치는 실측데이터의 평균값을 사용한다. 미분법은 Savitzky-Golay 알고리즘을 이용하여 적용하였으며, 사용된 필터값은 11, 필터 차수는 2차함수(quadratic filter)를 사용하였다. 이동평균(MA)의 경우 평활화 방식 중 하나로서, 중간값과 좌우의 값을 더하여 평균을 내는 방식을 적용하였다.
각 시료별 혼합율 판별모델 개발을 위하여 선형판별분석(LDA, linear discriminant analysis), 부분최소자승 판별분석(PLS-DA, partial least square discriminant analysis), 서포트벡터머신(SVM, support vector machine), 판별트리(CART, classification and regression tree) 및 램덤 포레스트(RF, random forest) 방법을 사용하였다(Gromski et al., 2014). LDA는 상관성이 높은 데이터가 군집을 형성하여 군집내 거리와 군집간 거리를 계산하여 가장 차이가 작은 것을 제1 LDA 값으로 선정한다. 주성분분석과 유사한 방법이지만 판별 성능을 평가할 수 있다는 점이 다르다. PLS-DA는 데이터를 mean centering한 후 사용자(supervisor)에 의해 부여된 카테고리 혹은 수치 클래스값에 따라 데이터를 판별한다. 모든 샘플에 사영한 값의 합(PRESS, PRESS = ∑i(yi - y︿l)2 yi는 입력변수, y︿l는 예측치)이 가장 작은 경우의 계수(beta coefficient)를 선택하여 판별에 사용한다. SVM에서 데이터 군집사이를 판별할 수 있는 직선 혹은 다중곡선을 초평면(hyper plane)이라고 하며, 각 군집에서 이 초평면 범위(마진) 안에 포함되는 샘플들을 서포트벡터(support vector)라고 한다. 여러 군집을 판별하는데 최적치를 설정하기 위해 초평면의 마진을 최대화할 수 있는 비용함수를 줄이는 기울기를 찾는 과정을 거치며, 비용함수 값이 0에 가까워질수록 좋은 결과를 나타낼 수 있다. 판별트리에서는 판별을 위해 선택한 각 변수를 노드(node)라고 하며, 각 노드에서 판별에 사용되는 경계치가 제시되며, 경계치에 의해 입력된 데이터가 클래스별로 갈라지게 된다. 랜덤 포레스트는 최근 판별 모델 개발에 많은 연구자들이 관심을 가지고 사용하고 있는 머신러닝의 한 방법이다. RF는 1) 다차원 데이터에 민감하지 않고, 2) 모델을 이용한 판별이 빠르고, 3) 계수의 조정이 크지 않으며, 4) 입력 데이터의 변수 중요도를 알 수 있다는 장점을 가지고 있다(Xia et al., 2018). 입력 데이터 중 선택된 변수를 이용하여 판별트리를 다수 만들어 예측값 중에서 가장 정확도가 큰 값을 선택하는 앙상블 방식을 차용한다(Breiman, 2001).
모델 개발을 위해 획득한 데이터는 모델훈련세트(N = 39,898)와 모델검증세트(N = 39,898)로 나누어 모델 개발 및 검증에 사용하였다. 개발된 모델 정확도를 확인하기 위해 판별분류표(confusion matrix)를 활용하였으며, 정확도(accuracy, A)와 카파계수(kappa coefficient, K)로 나타내었다. 정확도와 카파계수는 1에 가까울수록 모델의 판별성능이 우수함을 나타낸다. 3차원 영상데이터의 전처리, 스펙트럼 추출 및 개발된 판별모델의 영상적용은 Matlab (R2016a, The MathWorks Inc., Natick, MS, USA)을 사용하였으며, 스펙트럼 데이터의 분석 및 판별모델 개발은 R (ver.3.6.2, R Foundation for Statistical Computing, Vienna, Austria)과 다변량 분석 패키지를 활용하였다. 상기한 하이퍼데이터 전처리 및 판별모델 개발 과정은 Fig. 2a에 나타내었다. Fig. 2b에서는 스펙트럼으로 개발한 딥러닝 모델을 영상으로 나타내는 과정을 나타내었다(Chen et al., 2019). 1차원 CNN (convolutional neural network)판별 모델 개발을 위해 Python (www.python.org)에서 사용 가능한 딥러닝 학습용 오픈소스인 Tensorflow (www.tensorflow.org)와 Keras (keras.io)를 사용하였다.
Results and Discussion
시료 영상 및 시료별 스펙트럼
시료별 칼라영상을 Fig. 2에 나타내었다. 모든 시료는 3개씩 제작하여 초분광 영상을 획득하였으며, 획득한 영상은 전처리 과정을 통해 영상 보정을 거쳤다. 각 시료에서 스펙트럼을 추출하기 위하여 히스토그램 방식으로 선택된 ROI내부에서 스펙트럼을 추출하였다. 배경제거를 위해 공통적으로 10번째 파장영상(465 nm)을 사용했으며 배경 제거 및 마스크 제작을 위해 문턱치(TH)는 120을 사용하였다(Fig. 3). 문턱치를 계산하기 위해 465 nm 영상에서 가장 어두운 ROI의 스펙트럼과 배경의 히스토그램을 사용하였다(Fig. 3a). 465 nm 영상(Fig. 3b)에 문턱치를 적용하여 이차화한 마스크 영상은 Fig. 3c에 나타내었다. 획득한 각 시료별 스펙트럼의(420 - 730 nm) 평균 스펙트럼은 Fig. 4에 나타내었다. 전체 시료에서 9개의 관찰된 형광 피크는 다음과 같다(446.2, 465.4, 489.4, 518.2, 547, 580.6, 623.7, 671.7 nm). 관찰된 형광 피크 중 470 - 550 nm는 전분과 깊은 상관이 있는 것으로 보고 되었다(Liu et al., 2015). 특히, 617, 656, 681 nm는 안토시아닌과 카르테노이드(Merzlyak and Chivkunova, 2000; Siedliska et al., 2018), 곡물 가루의 형광 피크들은 주로 곡물의 주성분인 아밀로스, 아밀로펙틴 등 탄수화물을 구성하는 물질들과 연관이 있으며, 단백질, 지방, 및 수분과 밀접한 C-H 3차 오버톤(880 nm), O-H 2차 오버톤(750 - 900 nm) 및 N-H (962 - 1000 nm) 등이 발표되었다(Ma et al., 2019; Weng et al., 2020). 시료 중 쌀가루(C1R1 - C1R4)는 365 nm 자외선 광을 조사한 경우 465 nm와 489 nm 파장에서 강한 형광을 나타내고 있음을 관찰할 수 있다(Liu et al., 2015). 전분의 경우 밤전분(C2R3, 노란선)을 제외하고 유사한 피크를 보였으나 세기 차이가 있었다. 전분은 465 nm와 489 nm 부근에서의 피크 외에도 547, 580 nm 주위의 형광도 나타났는데 547 nm의 형광이 우세한 것으로 관찰된다.
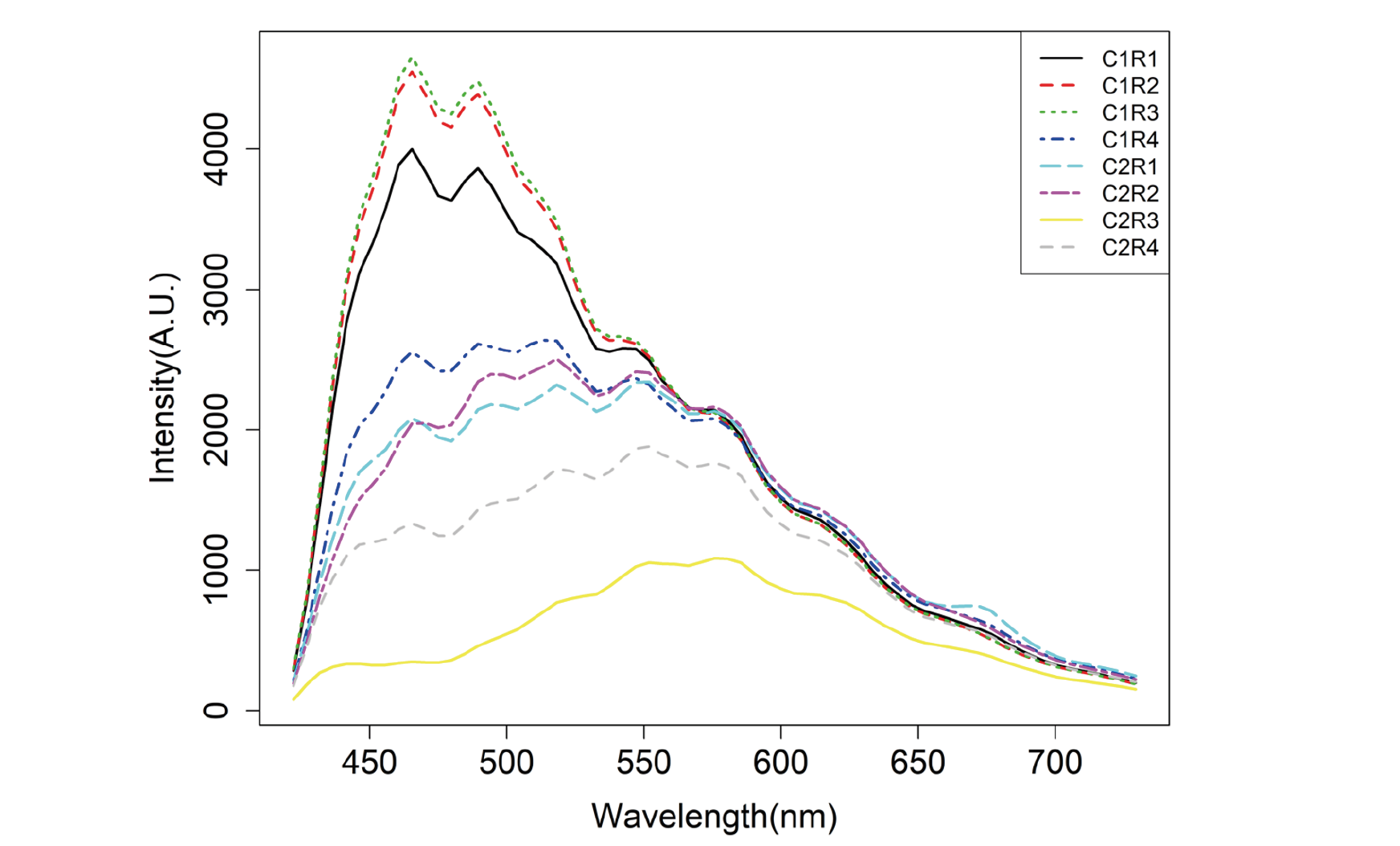
Fig. 4. Mean emission spectral data of 8 grain flours taken under 365 nm UV light illumination. C1R1, weak rice flour (WF); C1R2, mimyeon flour (MF1); C1R3, mimyeon flour (MF2); C1R4, strong rice flour (SF); C2R1, tapioca starch (TS); C2R2, sweatpotato starch (SS); C2R3, chestnut starch (CS); C2R4, mungbean starch (MS).
판별 모델 개발
초분광 영상에서 획득한 곡물 가루별 형광 스펙트럼으로 판별모델을 개발하기 위하여 사용한 모델별 판별결과를 Table 1에 나타내었다. 각 전처리별 모델의 성능은 정확도(A)와 카파계수(K)로 나타내었으며, 각 시료별로 판별결과도 각 행별로 나타내었다. 판별모델별 정확도에서는 LDA가 이동평균법(MA)으로 전처리한 경우에 가장 좋은 성능을 보였다(A = 0.9362, K = 0.9270). 쌀가루와 전분가루 판별에서는 좋은 성능을 보였으나 같은 품종의 다른 입자크기를 가진 미면의 판별성능은 다른 품종에 비해 떨어지는 것을 확인할 수 있었다. 곡물가루 8종류의 판별결과에서 미면(MF1, MF2)판별에는 SVM이 상대적으로 좋은 성능을 보였으며, 2차 미분(D2)으로 전처리한 경우 평균 판별성능은 0.7554 (MF1: 0.6666, MF2: 0.8443)로 가장 좋은 판별 결과를 나타내었다. 두 종류의 미면 시료만을 대상으로 판별한 경우에도 SVM과 2차 미분으로 전처리한 경우에 가장 좋은 판별 성능을 보였다(A = 0.8672, K = 0.7333). 미면 시료간에 60%의 입자의 크기(MF1: 128.3 ± 0.61 μm, MF2: 76.33 ± 1.02 μm) 차이가 있으나 물질의 화학적인 성분 및 농도의 차이에 따라 흡수한 광을 발광하는 형광의 특성으로 측정한 동일 물질의 입자의 크기 정밀 판별에는 정확도 0.75를 나타내었다. 동일 전처리 및 모델의 8 곡물 판별 결과가 0.9317에 비해 19% 낮은 정확도를 나타내었다. Fig. 5에서는 판별 모델 중 LDA를 사용하여 각 시료별로 군집 형성한 결과를 LD1과 LD2 공간에서 표현하였다. LD1은 62%, LD2는 24%의 판별력이 있는 것으로 나타나고 있으며 같은 품종이지만 입자 크기가 상이한 MF1와 MF2가 일부 중첩되는 것을 제외하고 대체로 시료별 군집이 좋은 것으로 관찰되었다(overall 86%).
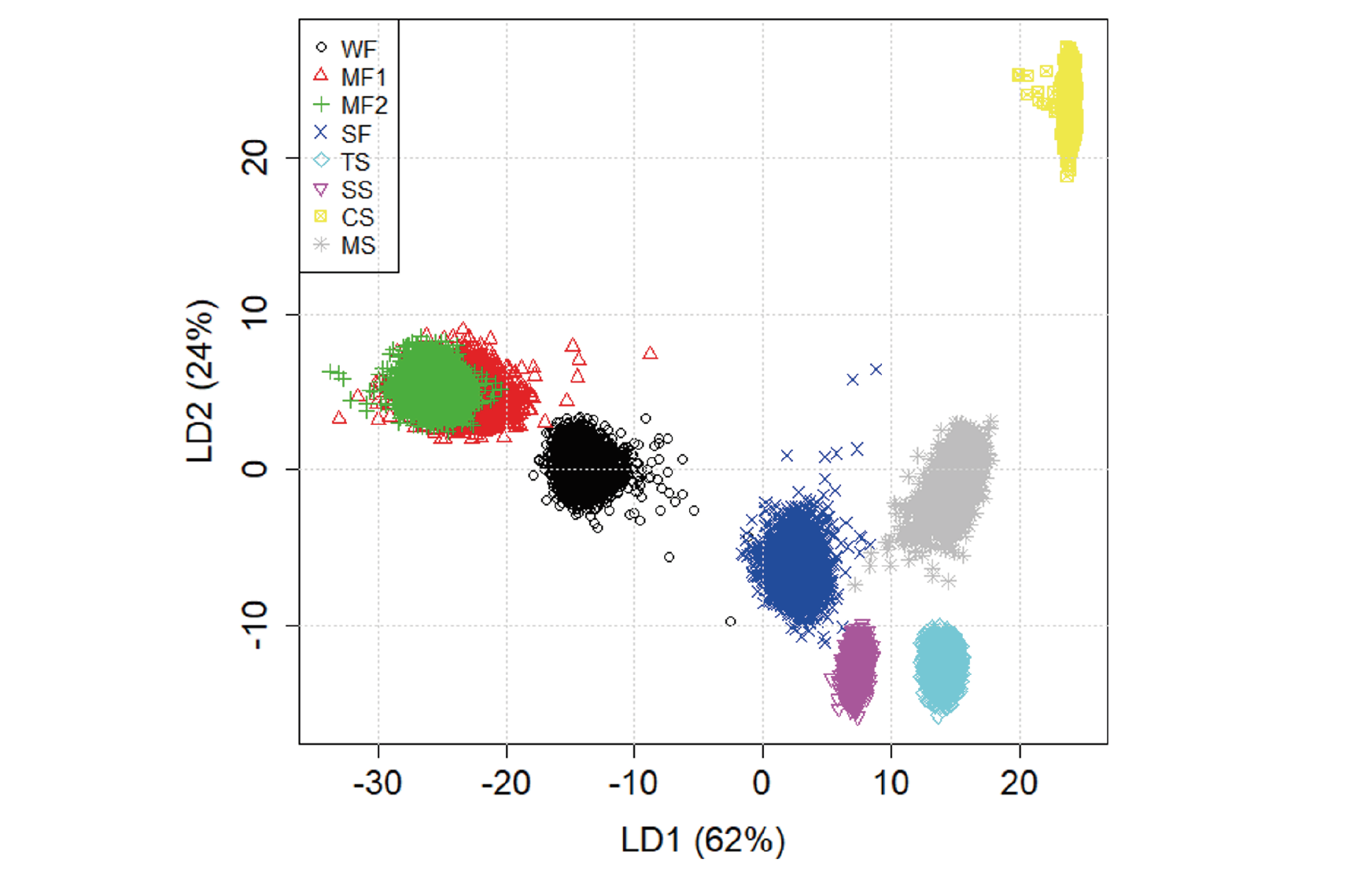
Fig. 5. Linear discriminant analysis (LDA) with moving average result in LD1 and LD2 space. LD1 has 62% and LD2 has 24% proportion of variance (overall 86%) in the space. WF, weak flour; MF1, mimyeon flour1; MF2, mimyeon flour2; SF, strong flour; TS, tapioca starch; SS, sweat potato starch; CS, chestnut starch; MS, mungbean starch.
판별 결과 영상 적용
초분광 영상데이터에서 추출한 분광정보로 개발한 판별모델을 초분광 영상에 적용하고 그 결과를 Fig. 6에 나타내었다. 결과의 표시를 용이하게 나타내기 위하여 판별결과에 따라 그레이 레벨의 의사색을(pseudo color)입혔다. LDA 계수를 추출하여 마스크 영상에 적용한 결과 Fig. 6a는 calibration 결과를 나타내며, Fig. 6b와 c는 모델 개발에 적용하지 않은 영상으로 판별 결과를 나타내었다. 이때 TH (120) 및 LDA 계수는 모델 개발에 사용한 값을 동일하게 사용하였다. 이로써 라이스페이퍼에 재료로 사용할 수 있는 곡물 가루의 초분광 형광데이터를 이용하여 스펙트럼뿐 아니라 영상으로도 각 시료별 판별이 가능함을 보였다. 다만 이 결과는 각 시료의 이화학 성분의 정량 측정없이 초분광 형광 데이터만으로 수행된 것이며 각 시료간에 이화학적인 판별을 위해서는 기기분석 결과를 첨부하여 화학 영상(chemical image) 분석도 가능할 것으로 판단된다.
판별 영상의 판별도를 명확하게 나타내기 위해 각 시료의 판정 결과를 히스토그램으로 표현하고 이를 결과분류표(confusion matrix)로 나타내었다(Fig. 7). LDA를 이용하여 개발된 판별 모델을 모델 개발에 사용하지 않은 2개의 시료에 적용한 결과 중에서 각 시료의 판별은 가능하였으나(A > 0.99) 입자크기는 다르지만 동일한 시료인 미면의 경우 A (MF1, MF2) = (0.69, 0.77) 및 (0.64, 0.7)으로 판별 결과를 보였다. 모델의 전체 정확도는 Fig. 7a에 적용한 모델 판별 결과 A = 0.93으로 나타났으며, 7b에 적용한 결과는 A = 0.91로 나타났다. 미면(MF1, MF2)의 판별 성능을 향상시키기 위하여 적용한 1차원 딥러닝의 결과를 결과분류표와 ROC 커브로 나타내었다(Fig. 8). 1차 CNN으로 판별한 결과는 A = 0.94로 나타났으며, MF1과 MF2의 판별은 0.72, 0.87로 향상된 것을 확인하였다.
Fig. 8. Confusion matrix (a) and receiver operating characteristic (b) based on 1D CNN. WF, weak flour; MF1, mimyeon flour1; MF2, mimyeon flour2; SF, strong flour; TS, tapioca starch; SS, sweat potato starch; CS, chestnut starch; MS, mungbean starch.
Conclusion
쌀 가공 식품 중 라이스페이퍼 제작을 위한 실험에 사용한 쌀 가루 및 전분 가루의 판별을 위해 초분광 형광데이터를 분석하고 모델을 개발하였다. 본 연구에서는 이화학적인 분석없이 파장대역 420 - 730 nm로 획득한 3차원 초분광 형광영상에서 스펙트럼을 추출하여 최적 판별모델을 개발하였다. 대표적인 선형판별모델인 LDA는 8개의 곡물 스펙트럼을 0.93이상의 정확도로 판별하였으나 입자 크기만 다른 미면 판별에는 0.73의 판별 정확도를 보였다. 미면만을 대상으로 분석한 결과는 이차 미분한 데이터를 SVM으로 판별(A = 0.86, K = 0.73)한 경우 가장 좋은 판별 결과를 나타내었다(데이터 미첨부). 미세 성분의 입자크기 선별의 경우 4,000 - 10,000 cm-1 (1,000 - 2,500 nm)의 FT-NIR (퓨리에 근적외분광)을 이용하여 크기가 6.9 - 21.7 μm의 항생제 원료로 사용되는 아목시실린 3수화물(amoxicillin trihydrate)을 판별한 바가 있다(Bittner et al., 2011). 형광을 위해 사용한 365 nm의 입사광에서 발광한 각 시료별 형광 스펙트럼이 판별에 효과적인 것으로 판단되며 향후 자동화 설비에서 정량공급 및 유해물질의 오염방지 등에 식품안전성 평가에도 적용될 수 있을 것으로 사료된다.