Introduction
The wine is a common consuming food product in our daily life and its quality measurement is of critical significance for wine classification and target marketing. The quality of wine influences several major aspects, including the wine quality rating, the reputation of the winery, and its profitability (Schamel and Anderson, 2003). The medicinal use of red wine has been estimated to dates back to 2200 BC, making it the oldest known medicine (Robinson, 2014).
Glycerol (C3H8O3), which is a by-product of alcoholic fermentation in wines, is a non-volatile compound that has no aromatic properties but contributes significantly to wine quality by providing sweetness and fullness. Glycerol influences the sensory characteristics of wine by contributing richness of taste (Nieuwoudt et al., 2002) and aroma perception (Lubbers et al., 2001). Several European countries already use glycerol content in wine for quality scaling. Generally, glycerol concentration in wine varies between 1 and 10 g·L-1 (Rankine and Bridson, 1971; Ough et al., 1972), although glycerol levels in red wine are higher when compared to white wines. The glycerol content in wine is set to be within a limit of 6 - 10 g per 100 g of alcohol, in accordance with the level of alcohol in wine (Popescu-Mitroi et al., 2014). Wines containing glycerol/alcohol below 6.5% are considered as fortified, while wines with a ratio exceeding 10% most likely contain artificially added glycerol (Beleniuc et al., 2013). The glycerol present in wine plays a very important physiological role in the human body, in that it esterifies blood-free fatty acids. Consequently, it can cause a decrease in cholesterol, which thickens blood vessel walls. In addition, the consumption of wines rich in glycerol favors the formation of prostacyclins, which are agents responsible for the dilation of blood vessels (i.e., reducing blood pressure) (Popescu-Mitroi et al., 2014). Thus, the quantification of glycerol in wines represents an important step in providing and facilitating wine quality certification.
Numerous analytical methods, including gas chromatography (Csutorás et al., 2014), electronic (Buratti et al., 2004) nose, high-performance liquid chromatography (Parpinello and Versari, 2000), gas chromatography-combustion-isotope ratio mass spectrometry (Calderone et al., 2004), have been commonly applied to the detection and quantification of glycerol concentration in wines to date. Further, analytical approaches employing immobilized enzymes in combination with various analytical techniques have been used for the determination of glycerol in wines (Nunes Fernandes et al., 2004). These conventional methods are very useful and reliable, however, they are also invasive, time-consuming, and not suitable for the increasing demands of real-time and on-line analyses of large quantities of samples. Thus, it is necessary to develop a method that would permit efficient and quantitative detection of glycerol concentration in red wines.
In the past, Raman spectroscopy (Martin et al., 2015) and near-infrared (NIR) spectroscopy (Ferrari et al., 2011) was used as a screening tool for the quality measurement of wines. Though, the following spectroscopic techniques have several analytical applications in the food and agriculture sectors (Hong et al., 2013; Amanah et al., 2020; Joshi et al., 2020, Faqeerzaada et al., 2020), still they suffered from certain limitations while dealing with fluorescence and overtones- combinations bands generating during spectral acquisitions. Thus, Fourier transform infrared spectroscopy is one of key solution while dealing with the former issues, by measuring fundamental vibrations of molecules. Fourier transform infrared (FT-IR) spectroscopy, is a non-destructive and label-free method used for the analysis of gases, liquids, and solids. In principle, infrared (IR) spectroscopy offers several advantages such as real-time monitoring and low maintenance when compared to gas chromatography. In addition, it is less expensive and provides more specific structural information than mass spectrometry (Doyle, 1992). The utility of FT-IR spectroscopy for the determination of glycerol concentration in wines was demonstrated in several studies employing immobilized enzymes in combination with various analytical techniques (Nunes Fernandes et al., 2004).
Although FT-IR spectroscopy has numerous applications in food quality measurement i.e benzene in edible oils (Joshi et al., 2019), protein and glucose of tubor and root flours determination using NIR and MIR spectroscopy (Masithoh et al., 2020) etc, the application of this technique to quality and authenticity measurements and the subsequent visual examination of the collected spectra do not provide sufficient information. Thus, it is necessary to exploit multivariate data analysis for extracting significant information from the obtained spectral data. In analytical chemistry, the purpose of multivariate analysis is data reduction, grouping, and classification, with the objective to develop a model that would specify the relationships that exists between variables (Seo et al., 2019). To this end, Lorber (1986) proposed the concept known as the net analyte signal (NAS), which describes the portion of the spectrum of a pure target analyte that is orthogonal to the background spectrum, which contains all the spectral components with the exception of the analyte. For dimensional reduction of the background spectra, combination of principal component analysis (PCA or PLS) is applied typically with NAS to reduce time and enhance performance (Lorber, 1986; Lorber et al., 1997).
The main objective of the present work is to demonstrate that the combination of FT-IR spectroscopy with NAS multivariate analysis can serve as a rapid, non-invasive, and high-throughput system for the quantitative determination of glycerol concentration in red wines non-destructively.
Materials and Methods
Sample preparation
Glycerol (> 98%) was purchased from Sigma Aldrich (St. Louis, MO, USA). Red wine with different brands (3 bottles from each brand) such as Shiraz, Merlot and Barbaresco from Australia, Chile and Italy were purchased from local wine shops in Korea and the samples were adulterated with different compositions of glycerol concentrations (0.1, 0.5, 1, 2, 3, 4, 5, 6, 7, 8, 9, 10, 11, 12, 13, 14, and 15% v·v-1) in a total volume of 40 mL. Further, the adulterated wine samples were transferred to individual snap-cap vials; then, the vortex mixer high-speed Vortex Gene2 (Scientific Industries, Inc., N.Y., USA) was used for 40 s to achieve the uniform solution. These ranges were selected in order to determine the adulterant detection limit in red wine. Ten replicates of each samples were selected from each of the eighteen adulterated groups with different amount of adulterant present. A total of 180 samples spectra were collected for each variety of wine (i.e., Shiraz, Merlot, and Barbaresco) from different countries, i.e., 540 samples were prepared for FT-IR spectroscopic measurement.
FT-IR spectroscopy
The collection of infrared spectra was performed using a Nicolet 6700 FT-IR spectrometer (Thermo Scientific Co., Waltham, MA, USA) configured in the attenuated total reflectance (ATR) sampling mode, and equipped with, a deuterated triglycine sulfate (DTGS) detector, and KBr beam splitter controlled by OMNIC software (Nicolet 6700, Thermo Fisher Scientific, Seoul, Korea). The spectra were collected separately for each sample at a wavelength range from 4,000 to 650 cm-1. For spectral measurement, a drop of wine was deposited on the surface of the diamond crystal sampling plate. The wine was removed using a dry tissue and the surface was rinsed first with alcohol and subsequently with water. Finally, the surface was dried with a clean tissue, in order to assure that no residue remain on the crystal plate from the previous sample and to acquire best sample spectra. Before the sample analysis, background scan was obtained once for pure wine and once for adulterated wine samples with an empty sample plate. In total, 32 successive scans were collected for each sample at 4 cm-1 intervals, and the averaged spectrum of each sample was used for analysis.
Data analysis
Spectral data are not suitable for direct analysis due to the generation of unwanted scattering and noise effects (due to the instrument and external factors) during spectral acquisition which affects the prediction performance of model. Preprocessing of the spectral data therefore plays a very important role in acquiring essential information from the measured sample (Faqeerzada et al., 2020). In this study, standard normal variate (SNV) was used as a preprocessing method for spectral analysis. SNV is normally considered as a scatter correction method that is designed to reduce the (physical) variability between samples caused by scatter or used to adjust for baseline shifts between samples (Rinnan et al., 2009). The basic format of SNV is given below:
= (1)
Here, a0 is the average value of the sample spectrum to be corrected, and a1 is the standard deviation of the sample spectrum. Data preprocessing represents the process of cleaning and preparing the data for classification or for prediction purposes. Data preprocessing represents the process of cleaning and preparing the data for classification or for prediction purposes.
In order to predict different concentrations of glycerol in red wine, net analyte signal (NAS) and hybrid linear analysis (HLA) algorithms were utilized as multivariate analysis methods. For the detailed description of HLA algorithm, the original article can be referred to (Goicoechea and Olivieri, 1999). The NAS extracts the part of a signal that is directly related to the concentration of the analyte of interest (Lorber, 1986). In this study, for calculating the NAS vector of each sample, a method introduced by Goicoechea and Olivieri (1999) and Marsili et al. (2003) was adopted. Fig. 1 illustrates the NAS concept through vector projection. In more detail, during the procedure a pure target analyte spectrum and background spectrum are collected, which consist of different types of variances, with the exception of the target analyte (Bai, 2010). NAS is a good calibration vector for quantitative analysis as it can demonstrate the spectral contributions based on background analysis. NAS allows the determination of various figures of merit (FOM) such as sensitivity (SEN), selectivity (SEL), limit of detection (LOD), and limit of quantification (LOQ) (Lorber, 1986; Lorber et al., 1997).
SEN normally characterizes the extent of signal variation as a function of analyte concentration, and higher sensitivity translates to a greater signal response following a change in concentration. In the NAS algorithm, the sensitivity of the NAS calibration model is estimated from Eq. (2):
= (2)
Here, bk is the vector of the final regression coefficients appropriate for component k. SEL is typically performed because it has the ability to quantify the analyte of interest accurately within the sample matrix. In multivariate calibration, SEL can be estimated from Eq. (3):
= (3)
Here, r* is the NAS vector and r is the sample spectrum. Further, LOD is the lowest analyte concentration that can be reliably distinguished from a sample with no analyte, whereas LOQ is the limit at which the differences between samples of two different concentrations can be observed (Lohumi et al., 2017). In the NAS algorithm, LOD and LOQ can be estimated from Eqs. (4) and (5):
(4)
(5)
Here, ‖ϵ‖ is used for measuring the instrumental noise, which may be evaluated by collecting several spectra of blank samples and calculating the corresponding standard deviation.
Further, for the evaluation of model performance, several parameters were utilized: coefficients of determination for calibration (Rc2) and validation (Rv2), root mean square errors of calibration (RMSEC) and validation (RMSEV), ratio of standard error of performance to standard deviation (RPD), and range error ratio (RER). Here, R2 is the key output of regression analysis. It is interpreted as the proportion of the variance in the dependent variable that is predictable from the independent variable and ranges from 0 to 1, where value close to 1 indicates a good fit. RMSE measures how much error there is between two datasets. In other words, RMSE compares a predicted value and an observed or known value. RPD attempts to scale the error in prediction based on the standard deviation of the property. If the error in estimation is large when compared to the standard deviation, then the model is not performing well. The RER value is used for assessing the quality of the model. Data analysis, including the chemometric analysis and computation, was performed using MATLAB version 7.0.4 (MathWorks, Inc., Natick, MA, USA).
Results and Discussion
Spectral interpretation
Fig. 2 shows the SNV preprocessed spectral profiles of wine data having three varieties adulterated with different glycerol concentration. For model development, only the fingerprint region (1,800 - 800 cm-1) was selected as it contains some peaks that are dependent on the glycerol concentration in red wine. From the list of main components in wine, glucose and fructose also play a vital role since they represent the total sugar content of wine, and show absorbance peaks in IR that are similar to those of organic acids (C-O and O-H), which are present at higher concentrations than sugars in dry wines (Dixit et al., 2005). Thus, several characteristic features were observed in the FT-IR spectra of the three different varieties of red wine spiked with glycerol between 1,600 and 800 cm-1. In particular, the peak within the region 1,420 - 1,320 cm-1 is associated with O-H bending, while the peak within the region 1,200 - 1,040 cm-1 represents C-O stretching. The region from 1,200 to 900 cm-1 contains the absorbance’s of several major components in wine i.e. ethyl alcohol and reducing sugars such as glucose and fructose (Okparanma and Mouazen, 2013). The peaks observed around 921, 993, 1,045 and 1,107 cm-1 corresponds to C-H and O-H vibrations respectively.
Hybrid linear analysis in the literature (HLA/GO) model for glycerol prediction
A multivariate calibration method named HLA/GO was adopted in order to predict the additional concentrations of glycerol in red wine based on the SNV pre-processed FT-IR spectra acquired under the spectral range of 1,800 - 800 cm–1. In order to obtain an optimum level of information and to avoid over-fitting, selection of an optimum number of factors is critical as it can facilitate better prediction capability. In this study, the optimum number of factors for HLA/GO algorithm was established to be five. These factors were selected on the basis of the lowest RMSE for the cross-validation set. Fig. 3c demonstrates the mean of the calculated NAS vector for each group in the validation set for three varieties of red wine, while Fig. 3a shows the spectrum of pure glycerol. It is apparent from these results that the NAS vector for pure red wine is almost flat, with no specific peaks. However, comparison with the spectrum of pure glycerol reveals that the NAS vector for the low concentration (0.1%) shows minor peaks in certain spectral regions that are sensitive to pure glycerol. Moreover, at higher concentrations (15%), the changes in the intensity of the NAS vector are proportional to the concentration of the analyte. The NAS regression plot shown in Fig. 3b was constructed by plotting the values of r* (NAS spectrum) as a function of s*(sensitivity vector). The obtained plot displays a good linear behavior for this dataset.
The coefficient of determination (R2) and root mean square error (RMSE) were used as statistical parameters for the evaluation of performance of the model (Basak et al., 2019). Fig. 4 shows the actual glycerol concentrations and the values predicted by the HLA/GO model for the various red wine samples, revealing an excellent agreement between the actual and predicted values. The R2 provides a measure of how close the data points are to the regression line of best fit. If the coefficient of determination (R2) is ± 1, the fit is perfect and the line describes the data accurately. If the value obtained for R2 is 0, this indicates that there is no linear correlation, and the straight line does not describe the data at all. For the development of the HLA/GO model, the datasets consisting of 540 samples was divided into calibration (378 samples) and validation (162 samples) sets shown in Table 1.
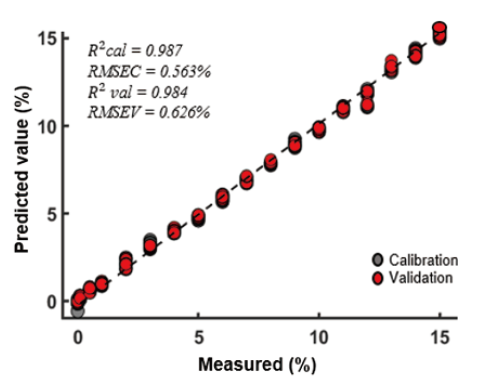
Fig. 4.Hybrid linear analysis in the literature (HLA/GO) graphs of actual versus predicted concentrations for calibration and validation plots of glycerol in red wine samples. Here, RMSEC indicates root mean square error of calibration, while RMSEV represents root mean square error of validation respectively.
Table 1. Datasets used during hybrid linear analysis in the literature (HLA/GO) model development for
glycerol adulteration prediction in ride wine samples.
![]() |
FT-IR, fourier transform infrared. |
Fig. 4 shows the relationships between pure glycerol and its actual concentrations in the tested samples at eighteen different concentrations, revealing a linear increase as the concentration increased from 0 to 15%. The calibration model afforded a very good correlation value (R2) of 0.987 and a low error (RMSEC) value of 0.563%, whereas the R2 and RMSEV values for the validation set were 0.984 and 0.626%, respectively. Thus, high R2 values and relatively low errors for the calibration and validation sets demonstrated that the combination of FT-IR spectroscopy with the HLA/GO-based multivariate calibration model is a robust tool for determining the concentration of glycerol added to red wine.
Further the beta-coefficient plot in Fig. 5, displays the spectral differences between various groups of samples. In multivariate analysis, beta plot is crucial for the localization of wavenumbers that provides useful information about the chemical features of compounds. The higher the beta value obtained, the greater the predicted value undergoes influence (Okparanma and Mouazen, 2013). Certain different types of important peaks were observed in the beta coefficients plot around 921, 993, 1,034 and 1,107 cm-1 which were under the similar spectral region sensitive to the glycerol a shown in (Fig. 3a). Thus, the beta-coefficient obtained from the HLA/GO method is attributable to the variation in glycerol concentration in red wine samples.
For comparison purposes, calculating figure of merit (FOM) is one of the common applications of NAS. Thus, the calculated FOM, including the values of SEN, SEL, LOD, and LOQ, for the HLA/GO method for glycerol concentration determination in red wine samples are summarized in Table 2. These parameters are particularly useful as they allow the performances of different models to be compared. Several approaches have been reported in the literature to obtaining the FOM for multivariate methods. The analytical SEN can be considered as the most useful parameter for method comparison, while the SEL measures the degree of overlap and shows the portion of the total signal that is not lost as a result of spectral overlap. The estimation of the instrumental noise, calculated from blank samples, allows us to determine the LOD.
Further, RER and RPD values were calculated for the prediction of glycerol concentration in red wine samples. Values for RER that are below 3 indicates that the model has a practical utility, whereas values between 3 and 10 suggest limited to good practical utility (Williams, 2001). In addition, the value of RPD considered for prediction accuracy is < 1.5, and indicates that the calibration is not usable, whereas values between 1.5 and 2.0 show the possibility of discriminating between low and high values. Finally, a value between 2.0 and 2.5 means that the model allows for approximate quantitative predictions. For values obtained between 2.5 and 3.0, and above 3.0, the prediction is classified as good and excellent, respectively. Thus, the calculated RER of 23.93 and RPD of 7.84 suggest that the established model produces very accurate estimations for the independent validation set. The present study attains a prediction accuracy value of 0.98 with lower standard error of prediction of 0.62% and higher LOD value of 0.14%. In comparison to a previous study (Nunes Fernandes et al., 2004) which although acquired higher prediction accuracy of 0.99, but it required complicated sample preparation, time consuming, expensive and is performed destructively. In this research, the obtained RER and RPD values contributes more advantages (mentioned above) in prediction analysis that were not calculated in the preceding research. Further, the advantages of the proposed method are rapid, easy sample preparation, inexpensive and presents a non-destructive measurement of glycerol concentration in red wine.
Conclusions
FT-IR spectroscopy coupled with HLA/GO analysis was applied for the quantitative analysis of glycerol concentration in red wine. The applied linear regression method demonstrated satisfying results. All datasets within the spectral ranges from 1,800 - 800 cm-1 were pre-treated by application of the SNV preprocessing method. The HLA/GO results for the calibration and validation sets revealed excellent accuracy (Rc2 = 0.987 and Rv2 = 0.984) and low error values (RMSEC = 0.563% and RMSEV = 0.626%) for a model based on 18 different glycerol concentrations in three varieties of wine. Hence, the obtained prediction results confirmed the potential of FT-IR spectroscopy when combined with multivariate analysis method (HLA/GO), for serving as a rapid, accurate and with a minimal sample preparation for predicting the concentration of glycerol in red wine. The developed model can be used to detect low adulteration percentage, with an LOD and LOQ of 0.14% and 0.47%. In future, this research will be further conducted for other different varieties of wine samples to examine the potential of the developed model for the determination of other possible wine adulterations in real time samples.
Authors Information
Rahul Joshi, https://orcid.org/0000-0002-5834-2893
Ritu Joshi, Chungnam National University, Department of Biosystems Machinery Engineering, Ph.D
Mohammad Akbar Faqeerzada, Chungnam National University, Department of Biosystems Machinery Engineering, Ph.D. student Hanim Z. Amanah, Chungnam National University, Department of Biosystems Machinery Engineering, Ph.D. student
J. Praveen Kumar, Chungnam National University, Department of Biosystems Machinery Engineering, Postdoctoral researcher
Geonwoo Kim, Environmental microbial and Food Safety Laboratory, Agricultural Research Service, United States Department of Agriculture, Postdoctoral researcher
Insuck Baek, Environmental microbial and Food Safety Laboratory, Agricultural Research Service, United States Department of Agriculture, Postdoctoral researcher
Eun-Sung Park, Chungnam National University, Department of Smart Agriculture Systems, Master student
Rudiati Evi Masithoh, Gadjah Mada University, Department of Agricultural and Biosystems Engineering, Faculty of Agricultural Technology, Professor
Byoung-Kwan Cho, https://orcid.org/0000-0002-8397-9853